Predictive Analytics in Energy Demand Forecasting with AI
Topic: AI Relationship Tools
Industry: Energy and Utilities
Discover how AI-driven predictive analytics can forecast energy demand optimize grid performance and enhance reliability in the energy sector
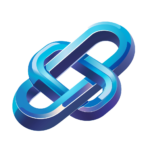
Predictive Analytics: Using AI to Forecast Energy Demand and Optimize Grid Performance
Understanding Predictive Analytics in the Energy Sector
Predictive analytics leverages advanced statistical techniques and machine learning algorithms to analyze historical data and forecast future outcomes. In the energy sector, this technology plays a crucial role in anticipating energy demand, enhancing grid performance, and ensuring a more reliable supply of electricity. By integrating artificial intelligence (AI) into predictive analytics, energy and utility companies can optimize their operations and improve customer satisfaction.
The Role of AI in Energy Demand Forecasting
AI-driven predictive analytics tools can process vast amounts of data from various sources, including weather patterns, historical consumption, and demographic information. This capability allows utilities to predict energy demand more accurately, leading to better resource allocation and grid management.
Key AI Techniques Used in Demand Forecasting
- Machine Learning Algorithms: These algorithms can identify patterns in historical data and make predictions based on those patterns. Techniques such as regression analysis, decision trees, and neural networks are commonly employed.
- Time Series Analysis: This approach analyzes data points collected or recorded at specific time intervals, enabling utilities to forecast future demand based on seasonal trends and historical usage.
- Natural Language Processing (NLP): NLP can be utilized to analyze customer feedback and social media sentiment, providing insights into consumer behavior that may impact energy demand.
AI-Driven Tools for Energy Demand Forecasting
Several AI-driven products are available to help energy and utility companies forecast demand and optimize grid performance. Some notable examples include:
1. IBM Watson for Energy
IBM Watson offers advanced analytics and AI capabilities that can help utilities predict energy consumption patterns. By integrating Watson into their operations, companies can analyze vast datasets to enhance forecasting accuracy and improve decision-making processes.
2. Siemens Spectrum Power
Siemens’ Spectrum Power is a comprehensive energy management system that utilizes AI and machine learning to optimize grid operations. It offers predictive analytics capabilities that help utilities manage demand response and maintain grid stability.
3. GE Digital’s Grid Solutions
GE Digital provides a suite of tools designed to enhance grid performance through predictive analytics. Their software solutions leverage AI to forecast demand and identify potential outages, enabling proactive maintenance and improved reliability.
Optimizing Grid Performance with AI
In addition to demand forecasting, AI can significantly enhance grid performance through real-time monitoring and predictive maintenance. By analyzing data from smart meters, sensors, and IoT devices, utilities can gain insights into grid health and performance.
Benefits of AI-Driven Grid Optimization
- Enhanced Reliability: Predictive maintenance powered by AI can identify potential equipment failures before they occur, reducing downtime and improving service reliability.
- Improved Efficiency: AI algorithms can optimize energy distribution, ensuring that electricity is delivered where it is needed most, thereby reducing waste and costs.
- Informed Decision-Making: Real-time data analysis allows utilities to make informed decisions regarding grid management, ultimately leading to better energy distribution and customer satisfaction.
Conclusion
As the energy landscape continues to evolve, the integration of AI and predictive analytics will be essential for utilities seeking to optimize performance and meet growing demand. By leveraging advanced tools and technologies, energy companies can enhance their forecasting capabilities, improve grid reliability, and ultimately deliver better service to their customers. The future of energy management lies in the ability to harness the power of AI, making predictive analytics a critical component of the energy and utilities sector.
Keyword: AI predictive analytics energy demand