Machine Learning in Automotive Supply Chain Optimization
Topic: AI Research Tools
Industry: Automotive
Discover how machine learning optimizes automotive supply chains through demand forecasting inventory management and supplier risk assessment for enhanced efficiency
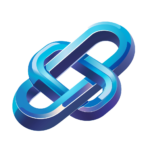
Machine Learning Applications for Optimizing Automotive Supply Chains
Understanding the Role of Machine Learning in Supply Chain Management
The automotive industry is characterized by its complexity and the necessity for efficiency. With the increasing demand for vehicles and the need for rapid production cycles, companies are turning to machine learning (ML) to optimize their supply chains. Machine learning, a subset of artificial intelligence (AI), offers powerful tools that can analyze vast amounts of data, identify patterns, and provide actionable insights, thus enhancing decision-making processes.Key Areas of Application
Demand Forecasting
One of the most significant applications of machine learning in automotive supply chains is demand forecasting. Traditional forecasting methods often rely on historical data and can be inaccurate due to unforeseen market changes. Machine learning algorithms, such as regression models and time series forecasting, can process real-time data, including market trends, consumer behavior, and economic indicators, to provide more accurate demand predictions.Inventory Management
Effective inventory management is crucial in minimizing costs and ensuring product availability. Machine learning can optimize inventory levels by predicting the optimal stock needed at various points in the supply chain. Tools such as IBM Watson Supply Chain use AI to analyze inventory data, helping companies to reduce excess stock and mitigate the risk of stockouts.Supplier Selection and Risk Management
Selecting the right suppliers and managing risks associated with them is another area where machine learning can be beneficial. AI-driven tools can evaluate supplier performance based on historical data, quality metrics, and delivery timelines. For instance, SAP Ariba utilizes machine learning algorithms to assess supplier reliability and predict potential disruptions, allowing automotive companies to make informed sourcing decisions.AI-Driven Tools for Automotive Supply Chains
1. IBM Watson Supply Chain
IBM Watson Supply Chain leverages AI to provide insights into supply chain operations. By analyzing data from various sources, it helps businesses anticipate disruptions and optimize logistics. The platform’s machine learning capabilities allow for continuous improvement in forecasting and inventory management.2. SAP Integrated Business Planning (IBP)
SAP IBP combines machine learning with advanced analytics to enhance supply chain planning. It enables automotive manufacturers to create more accurate demand forecasts and optimize production schedules. The tool also provides scenario planning features, allowing companies to assess the impact of different variables on their supply chain.3. Llamasoft Supply Chain Guru
Llamasoft Supply Chain Guru is a comprehensive supply chain modeling tool that incorporates machine learning to improve decision-making. It allows automotive companies to simulate various supply chain scenarios and evaluate the impact of changes in demand, supply, and logistics. This capability is crucial for developing resilient supply chain strategies.Implementation Strategies
Data Integration
To effectively implement machine learning solutions, automotive companies must first ensure robust data integration across their supply chain systems. This involves consolidating data from various sources, such as ERP systems, CRM platforms, and IoT devices, to create a comprehensive dataset for analysis.Collaboration with AI Experts
Collaborating with AI experts and data scientists can facilitate the successful implementation of machine learning technologies. These professionals can help automotive companies identify the right algorithms, interpret data insights, and develop tailored solutions that align with specific business objectives.Continuous Learning and Adaptation
Machine learning models require continuous learning and adaptation to remain effective. Automotive companies should establish processes for regularly updating their models with new data and insights. This iterative approach ensures that the supply chain remains agile and responsive to changing market conditions.Conclusion
The integration of machine learning into automotive supply chains presents significant opportunities for optimization and efficiency. By leveraging AI-driven tools, companies can improve demand forecasting, enhance inventory management, and mitigate supplier risks. As the automotive industry continues to evolve, the strategic implementation of machine learning will be essential for maintaining a competitive edge in an increasingly complex landscape.Keyword: automotive supply chain optimization