Machine Learning Innovations in Biodiversity Monitoring and Conservation
Topic: AI Research Tools
Industry: Environmental Sciences
Discover how machine learning is transforming biodiversity monitoring and conservation with innovative tools and strategies for effective ecosystem protection
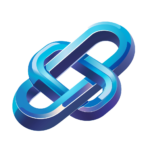
How Machine Learning is Transforming Biodiversity Monitoring and Conservation
Introduction to Machine Learning in Environmental Sciences
Machine learning (ML), a subset of artificial intelligence (AI), is making significant strides in various fields, including environmental sciences. Its ability to analyze vast amounts of data and identify patterns is proving invaluable in biodiversity monitoring and conservation efforts. By harnessing the power of ML, researchers and conservationists can gain deeper insights into ecosystems, enabling more effective strategies for preserving biodiversity.
The Role of Machine Learning in Biodiversity Monitoring
Biodiversity monitoring involves the systematic observation and assessment of various species and their habitats. Traditional methods often rely on manual surveys, which can be time-consuming and sometimes inaccurate. Machine learning offers innovative solutions to enhance these processes.
Data Collection and Analysis
One of the primary challenges in biodiversity monitoring is the sheer volume of data collected from various sources, such as satellite imagery, camera traps, and ecological surveys. ML algorithms can process this data efficiently, identifying species and assessing their populations with greater accuracy.
Example Tools and Applications
- Google Earth Engine: This platform allows researchers to access and analyze satellite imagery over time. By using ML algorithms, users can track changes in land use and habitat loss, helping to identify areas in need of conservation.
- Wildlife Insights: An AI-driven platform that analyzes camera trap images to identify species automatically. By using deep learning techniques, Wildlife Insights significantly reduces the time required for data processing, enabling quicker decision-making for conservation efforts.
Enhancing Conservation Strategies with AI
In addition to monitoring, machine learning plays a critical role in developing and implementing conservation strategies. By predicting trends and modeling ecological outcomes, AI can help conservationists make informed decisions.
Predictive Modeling
Machine learning algorithms can analyze historical data to predict future biodiversity trends. This capability allows conservationists to anticipate potential threats, such as climate change impacts or habitat destruction, and to devise proactive strategies to mitigate these risks.
Example Tools and Applications
- MaxEnt: A popular tool for species distribution modeling, MaxEnt uses presence-only data to predict the suitable habitat for various species. This information can guide conservation efforts by highlighting critical areas for protection.
- IBM Watson: With its advanced data analytics capabilities, IBM Watson can assist in analyzing ecological data to identify patterns and correlations that may not be evident through traditional analysis methods.
Challenges and Considerations
While the integration of machine learning into biodiversity monitoring and conservation presents numerous advantages, it is not without challenges. Data quality, availability, and the need for interdisciplinary collaboration are critical factors that must be addressed to maximize the potential of AI in this field.
Data Quality and Accessibility
High-quality data is essential for training effective machine learning models. Ensuring that data is accurate, relevant, and accessible is crucial for the success of AI-driven conservation efforts. Collaboration between researchers, governments, and organizations can help improve data-sharing practices.
Interdisciplinary Collaboration
The successful application of machine learning in biodiversity conservation requires collaboration across various disciplines, including ecology, computer science, and data analytics. By fostering partnerships and sharing expertise, stakeholders can enhance the effectiveness of conservation strategies.
Conclusion
Machine learning is revolutionizing the way we monitor and conserve biodiversity. By leveraging AI-driven tools and applications, researchers and conservationists can gain critical insights, predict trends, and develop more effective strategies to protect our planet’s diverse ecosystems. As technology continues to evolve, the potential for machine learning in environmental sciences will only expand, offering new opportunities for safeguarding biodiversity for future generations.
Keyword: machine learning biodiversity conservation