SolarWinds Network Performance Monitor
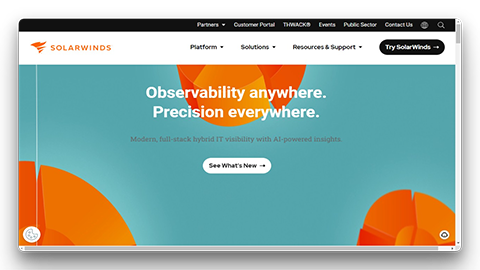
SolarWinds Network Performance Monitor (NPM) is a robust network monitoring solution designed for IT teams seeking to enhance and optimize network performance across various environments. It offers real-time visibility into network health and performance, leveraging advanced analytics to quickly identify and resolve issues. NPM is well-suited for multi-vendor environments, providing comprehensive support that includes customizable alerts and dashboards tailored to specific management needs. While it excels in delivering detailed insights and proactive notifications, users may find the initial setup and configuration complex, and advanced features may come at a higher cost. Overall, SolarWinds NPM is an effective tool for organizations aiming to maintain efficient network operations.