AI Search Tools Balancing Renewable Integration and Grid Stability
Topic: AI Search Tools
Industry: Energy and Utilities
Discover how AI search tools enhance grid stability by optimizing renewable energy integration through predictive analytics real-time monitoring and demand response management
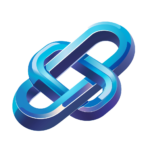
The Role of AI Search in Balancing Renewable Integration and Grid Stability
Introduction to AI Search in Energy and Utilities
As the energy sector increasingly embraces renewable sources, the challenge of maintaining grid stability becomes paramount. The integration of artificial intelligence (AI) search tools into energy management systems offers innovative solutions to this pressing issue. These tools not only enhance operational efficiency but also facilitate the seamless integration of renewable energy sources into existing grid infrastructures.
Understanding the Challenges of Renewable Integration
Renewable energy sources, such as solar and wind, are inherently variable and intermittent. This variability can lead to fluctuations in power supply, which, if not managed effectively, can jeopardize grid stability. Traditional grid management methods often struggle to cope with these fluctuations, resulting in inefficiencies and potential outages. AI search tools can play a critical role in addressing these challenges by providing real-time data analysis and predictive insights.
How AI Search Tools Enhance Grid Stability
AI search tools utilize advanced algorithms and machine learning techniques to analyze vast amounts of data from various sources. This capability allows energy providers to make informed decisions that enhance grid reliability. Here are several ways in which AI search tools contribute to balancing renewable integration and grid stability:
1. Predictive Analytics
AI-driven predictive analytics can forecast energy demand and supply trends with remarkable accuracy. By analyzing historical data, weather patterns, and consumption behaviors, these tools enable utilities to anticipate fluctuations and adjust their operations accordingly. For example, tools like AutoGrid employ AI algorithms to optimize energy distribution based on predicted demand, ensuring that supply meets consumption without overloading the grid.
2. Real-Time Monitoring and Control
AI search tools provide real-time monitoring of grid conditions, allowing operators to identify potential issues before they escalate. Systems such as Siemens’ Spectrum Power utilize AI to continuously analyze grid performance, providing operators with actionable insights to manage grid stability effectively. This proactive approach minimizes the risk of outages and enhances overall reliability.
3. Demand Response Management
AI search tools facilitate demand response strategies by analyzing consumer behavior and energy usage patterns. By implementing AI-driven platforms such as EnerNOC, utilities can incentivize customers to reduce or shift their energy usage during peak periods, thus alleviating stress on the grid. This not only helps in maintaining stability but also promotes energy efficiency.
4. Integration of Distributed Energy Resources (DERs)
The rise of distributed energy resources, including rooftop solar panels and battery storage systems, presents both opportunities and challenges for grid management. AI search tools can optimize the integration of these resources by intelligently managing their output and coordinating with the central grid. Solutions like Enel X leverage AI to optimize the performance of DERs, ensuring they contribute positively to grid stability.
Case Studies: Successful Implementations of AI Search Tools
Case Study 1: Pacific Gas and Electric (PG&E)
PG&E has successfully integrated AI search tools to enhance its grid management capabilities. By deploying machine learning algorithms to analyze data from various sources, PG&E has improved its ability to predict demand spikes and manage renewable energy inputs, resulting in a more stable grid and reduced operational costs.
Case Study 2: Duke Energy
Duke Energy implemented AI-driven analytics to optimize its renewable energy integration strategies. The company utilized AI to analyze weather patterns and energy consumption trends, allowing for better forecasting and more effective management of its renewable assets. This initiative has led to significant improvements in grid reliability and operational efficiency.
Conclusion
The integration of AI search tools in the energy sector is not just a trend; it is a necessity for achieving a stable and reliable grid in the face of increasing renewable energy adoption. By leveraging predictive analytics, real-time monitoring, demand response management, and effective integration of distributed resources, utilities can enhance grid stability while promoting sustainability. As the energy landscape continues to evolve, the role of AI will undoubtedly become more critical in balancing the complexities of renewable integration and grid management.
Keyword: AI search tools for grid stability