AI Driven Threat Detection for Agricultural Data Security
Topic: AI Security Tools
Industry: Agriculture
Discover how AI-driven threat detection enhances data security in farm management systems ensuring the protection of sensitive agricultural information.
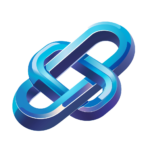
Safeguarding Agricultural Data: AI-Driven Threat Detection for Farm Management Systems
The Importance of Data Security in Agriculture
As the agricultural sector increasingly adopts digital technologies, the protection of sensitive data becomes paramount. Farm management systems, which collect and analyze vast amounts of information related to crop yields, soil health, and resource management, are particularly vulnerable to cyber threats. Safeguarding this data is essential not only for operational efficiency but also for maintaining trust among stakeholders.
Artificial Intelligence: A Game Changer in Data Security
Artificial intelligence (AI) is revolutionizing the way agricultural businesses approach data security. By leveraging AI-driven tools, farmers and agribusinesses can enhance their threat detection capabilities, ensuring that their data remains secure from unauthorized access and cyberattacks.
How AI Enhances Threat Detection
AI technologies can analyze large datasets in real-time, identifying patterns and anomalies that may indicate potential security threats. This proactive approach allows for quicker response times and minimizes the risk of data breaches. Key AI-driven functionalities include:
- Anomaly Detection: AI algorithms can establish baseline behaviors for data access and usage, alerting operators to any deviations that may signify a security threat.
- Predictive Analytics: By analyzing historical data, AI can forecast potential vulnerabilities and recommend preventive measures.
- Automated Response: AI systems can initiate automated responses to certain threats, mitigating risks without human intervention.
AI-Driven Tools for Agricultural Data Security
Several AI-driven products and tools are available to enhance the security of farm management systems. Below are some notable examples:
1. IBM Watson for Agriculture
IBM Watson offers a suite of AI tools that can be integrated into farm management systems to enhance data security. Its machine learning capabilities allow for real-time monitoring of data access patterns, helping to identify unusual activities that may indicate a security breach.
2. Microsoft Azure FarmBeats
Microsoft Azure FarmBeats leverages AI and IoT technologies to provide insights into farm operations. Its security features include data encryption and access controls, ensuring that sensitive information is protected against unauthorized access.
3. AgriWebb
AgriWebb is a farm management software that incorporates AI to enhance operational efficiency and data security. The platform includes features for tracking user activity and implementing security protocols based on AI-driven analytics.
4. CropX
CropX utilizes AI to optimize irrigation and soil management. The platform also includes robust security measures that protect agricultural data from external threats, ensuring that farmers can focus on productivity without worrying about data breaches.
Implementing AI Security Tools in Farm Management Systems
To effectively implement AI-driven security tools within farm management systems, agricultural businesses should consider the following steps:
1. Assess Current Security Posture
Conduct a thorough assessment of existing data security measures to identify vulnerabilities and areas for improvement.
2. Choose the Right AI Tools
Select AI-driven tools that align with specific security needs and operational goals. Consider factors such as scalability, ease of integration, and user-friendliness.
3. Train Staff on AI Technologies
Provide training for staff members to ensure they are familiar with the AI tools being implemented and understand their role in maintaining data security.
4. Continuously Monitor and Update Security Protocols
Data security is an ongoing process. Regularly monitor AI systems for performance and security effectiveness, and update protocols as needed to address emerging threats.
Conclusion
As the agricultural sector continues to embrace digital transformation, the need for robust data security measures becomes increasingly critical. Implementing AI-driven threat detection tools within farm management systems not only enhances data protection but also fosters a culture of security awareness among agricultural businesses. By proactively safeguarding their data, farmers can focus on what they do best: cultivating sustainable and productive agricultural practices.
Keyword: AI threat detection in agriculture