AI Enhanced Quality Control with Vision Systems for Manufacturing
Topic: AI Self Improvement Tools
Industry: Manufacturing and Industrial Automation
Discover how AI-enhanced vision systems revolutionize quality control in manufacturing ensuring flawless production and superior product quality for competitive advantage
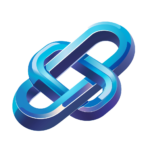
AI-Enhanced Quality Control: Cutting-Edge Vision Systems for Flawless Production
The Evolution of Quality Control in Manufacturing
In recent years, the manufacturing sector has witnessed a significant transformation driven by advancements in artificial intelligence (AI) and automation technologies. Traditional quality control processes, often reliant on manual inspections and human judgment, are increasingly being augmented by AI-enhanced systems that promise higher accuracy, efficiency, and consistency. These innovations are not merely incremental improvements; they represent a paradigm shift in how quality assurance is approached across industries.
Understanding AI-Driven Vision Systems
At the heart of this evolution are AI-driven vision systems that utilize machine learning algorithms, computer vision, and data analytics to monitor production processes in real-time. These systems are designed to detect defects, measure product specifications, and ensure compliance with quality standards far more effectively than human inspectors.
Key Components of AI Vision Systems
- Machine Learning Algorithms: These algorithms allow the system to learn from data patterns, improving their accuracy over time.
- High-Resolution Cameras: Equipped with advanced imaging technology, these cameras capture detailed images of products on the assembly line.
- Data Analytics: AI systems analyze the captured data to identify anomalies and trends, providing actionable insights for quality improvement.
Implementing AI in Quality Control
Integrating AI into quality control processes involves several key steps:
1. Assessing Current Processes
Manufacturers must begin by evaluating their existing quality control procedures to identify areas where AI can add value. This assessment should focus on identifying bottlenecks, common defects, and the effectiveness of current inspection methods.
2. Selecting the Right Tools
Choosing the appropriate AI-driven tools is crucial. Some notable examples include:
- Cognex Vision Systems: Known for their powerful image processing capabilities, Cognex systems can identify defects in real-time, ensuring immediate corrective actions.
- Keyence Inspection Systems: These systems utilize advanced laser and imaging technology to conduct precise measurements and inspections, enhancing overall product quality.
- Siemens MindSphere: A cloud-based IoT operating system that connects machines and physical infrastructure to harness data analytics for predictive maintenance and quality assurance.
3. Training and Calibration
Once the tools are selected, it is essential to train staff on how to operate them effectively. This includes calibrating the systems to recognize specific defects and adjusting parameters based on production needs.
4. Continuous Monitoring and Improvement
AI systems should be continuously monitored and refined to adapt to new challenges and improve detection capabilities. Regular updates and retraining of machine learning models are necessary to maintain high performance.
Case Studies: Success Stories in AI-Enhanced Quality Control
Several companies have successfully implemented AI-driven vision systems to enhance their quality control processes:
Example 1: Tesla
Tesla employs advanced AI vision systems in its manufacturing plants to monitor vehicle assembly. By utilizing machine learning algorithms, the company has significantly reduced defects in paint quality and assembly precision, leading to higher customer satisfaction.
Example 2: Coca-Cola
Coca-Cola has integrated AI-powered inspection systems in its bottling plants. These systems ensure that every bottle meets strict quality standards by detecting issues such as label misalignment and fill levels, resulting in minimized waste and enhanced product integrity.
The Future of Quality Control
The adoption of AI-enhanced quality control systems is not just a trend; it is a necessary evolution for manufacturers aiming to remain competitive in a rapidly changing marketplace. As technology continues to advance, we can expect even more sophisticated tools that leverage AI to drive quality assurance.
Conclusion
AI-enhanced vision systems represent a cutting-edge solution for achieving flawless production in the manufacturing sector. By implementing these technologies, companies can not only improve their quality control processes but also foster a culture of continuous improvement and innovation. As we look to the future, the integration of AI in manufacturing will undoubtedly pave the way for enhanced efficiency, reduced costs, and superior product quality.
Keyword: AI quality control systems