Machine Learning for Supply Chain Optimization and Efficiency
Topic: AI Self Improvement Tools
Industry: Manufacturing and Industrial Automation
Discover how machine learning transforms supply chain optimization by enhancing forecasting improving inventory management and boosting operational efficiency
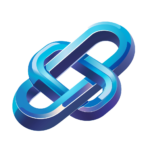
Machine Learning in Supply Chain Optimization: Streamlining Industrial Processes
Understanding the Role of Machine Learning
Machine learning (ML) has emerged as a pivotal force in transforming supply chain management across various industries. By leveraging vast amounts of data, ML algorithms can identify patterns, predict outcomes, and optimize processes, thus enhancing efficiency and reducing costs. In the realm of manufacturing and industrial automation, these capabilities are particularly invaluable.
Key Benefits of Machine Learning in Supply Chain Management
Implementing machine learning in supply chain optimization offers several key advantages:
- Enhanced Forecasting: Machine learning algorithms can analyze historical data to predict demand more accurately, allowing manufacturers to adjust production schedules accordingly.
- Improved Inventory Management: By predicting stock levels and turnover rates, ML can help businesses maintain optimal inventory levels, reducing excess stock and minimizing stockouts.
- Increased Operational Efficiency: Automated processes powered by AI can streamline workflows, reduce manual errors, and improve overall productivity.
- Cost Reduction: By optimizing various aspects of the supply chain, companies can significantly lower operational costs and enhance profitability.
Implementing Machine Learning in Supply Chain Processes
To effectively integrate machine learning into supply chain operations, organizations can follow a structured approach:
1. Data Collection and Preparation
The first step involves gathering relevant data from various sources, including sales records, inventory levels, supplier performance, and market trends. This data must then be cleaned and formatted to ensure accuracy and consistency.
2. Choosing the Right Algorithms
Selecting appropriate machine learning algorithms is crucial for achieving desired outcomes. Common algorithms used in supply chain optimization include:
- Regression Analysis: Useful for demand forecasting and price optimization.
- Classification Algorithms: Help in categorizing products based on sales performance.
- Clustering Techniques: Identify similarities among products or suppliers to enhance decision-making.
3. Implementation of AI-Driven Tools
Several AI-driven products and tools are available to facilitate the integration of machine learning into supply chain processes:
IBM Watson Supply Chain
IBM Watson Supply Chain utilizes AI and machine learning to provide real-time insights into supply chain operations. It helps businesses predict disruptions, optimize inventory levels, and improve supplier collaboration.
Microsoft Azure Machine Learning
Microsoft Azure offers a robust platform for building, training, and deploying machine learning models. Its capabilities can be harnessed to enhance demand forecasting and inventory management in manufacturing.
Kinaxis RapidResponse
Kinaxis provides a cloud-based supply chain management solution that leverages machine learning to improve planning and execution. It offers features like scenario simulation and real-time analytics to support decision-making.
Case Studies: Successful Implementations
Real-world examples illustrate the effectiveness of machine learning in supply chain optimization:
Case Study 1: Unilever
Unilever implemented machine learning algorithms to enhance its demand forecasting capabilities. By analyzing historical sales data and external factors, the company improved its forecasting accuracy by 50%, leading to better inventory management and reduced waste.
Case Study 2: Amazon
Amazon utilizes advanced machine learning models to optimize its logistics and supply chain operations. The company’s AI-driven systems analyze customer behavior to predict demand and streamline its fulfillment processes, resulting in faster delivery times and improved customer satisfaction.
Conclusion
As industries continue to evolve, the role of machine learning in supply chain optimization becomes increasingly vital. By adopting AI self-improvement tools, manufacturers can streamline their operations, enhance decision-making, and ultimately drive profitability. Embracing these technologies not only positions companies for success in today’s competitive landscape but also prepares them for future advancements in industrial automation.
Keyword: machine learning supply chain optimization