AI Speech Tools Enhancing Predictive Maintenance in Renewable Energy
Topic: AI Speech Tools
Industry: Energy
Discover how AI speech tools enhance predictive maintenance in renewable energy facilities boosting efficiency and reducing downtime for wind and solar operations
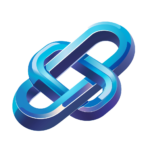
AI Speech Tools for Predictive Maintenance in Renewable Energy Facilities
Understanding Predictive Maintenance
Predictive maintenance is a proactive approach to asset management that leverages data analysis to predict when equipment failures might occur. In the context of renewable energy facilities, such as wind farms and solar power plants, this methodology is crucial for maximizing operational efficiency and minimizing downtime. By employing advanced technologies, organizations can enhance their maintenance strategies, ensuring that renewable energy systems operate at peak performance.
The Role of AI Speech Tools
Artificial intelligence (AI) has transformed various industries, and the renewable energy sector is no exception. AI speech tools are emerging as a vital component in predictive maintenance, enabling professionals to streamline communication, access information quickly, and make data-driven decisions. These tools utilize natural language processing (NLP) and machine learning algorithms to interpret and respond to human speech, thus facilitating interactions between technicians and maintenance systems.
Benefits of AI Speech Tools
- Enhanced Communication: AI speech tools enable technicians to communicate with maintenance systems using voice commands, reducing the need for manual data entry and allowing for hands-free operation.
- Real-Time Data Access: Technicians can retrieve critical information about equipment status, maintenance schedules, and operational metrics through voice queries, improving response times and decision-making.
- Improved Training and Support: Speech recognition technology can assist in training new employees by providing verbal instructions and troubleshooting guidance, thus enhancing workforce competency.
Implementing AI Speech Tools in Renewable Energy Facilities
To effectively implement AI speech tools in predictive maintenance, organizations should consider the following steps:
1. Assess Current Systems
Evaluate existing maintenance processes and identify areas where AI speech tools can add value. This may include analyzing data flows, communication patterns, and technician workflows.
2. Choose the Right Tools
Select AI speech tools that align with organizational needs. Some notable products include:
- IBM Watson: This AI platform offers speech recognition capabilities that can be integrated into maintenance management systems, allowing technicians to access information and log issues through voice commands.
- Google Cloud Speech-to-Text: This service enables real-time transcription of spoken language into text, making it easier for technicians to document maintenance activities and communicate findings.
- Amazon Alexa for Business: By deploying Alexa-enabled devices, organizations can facilitate hands-free communication, enabling technicians to query equipment data and receive updates without interrupting their tasks.
3. Train Staff
Provide comprehensive training for staff on how to utilize AI speech tools effectively. This includes familiarizing them with voice commands, troubleshooting procedures, and best practices for integrating speech tools into their daily routines.
4. Monitor and Optimize
After implementation, continuously monitor the performance of AI speech tools and gather feedback from users. Use this data to make necessary adjustments and optimize the tools for better efficiency and effectiveness.
Case Studies: Successful Implementations
Several renewable energy facilities have successfully integrated AI speech tools into their predictive maintenance strategies:
Case Study 1: Wind Farm Operations
A leading wind farm operator implemented IBM Watson to enhance their maintenance processes. Technicians began using voice commands to check turbine statuses and log maintenance requests, resulting in a 20% reduction in downtime and improved response times to equipment issues.
Case Study 2: Solar Power Plant
At a large solar power facility, Google Cloud Speech-to-Text was adopted to streamline communication between field technicians and the control center. This integration allowed for real-time updates on equipment performance, leading to a significant increase in overall operational efficiency.
Conclusion
AI speech tools represent a transformative opportunity for predictive maintenance in renewable energy facilities. By enhancing communication, improving data accessibility, and streamlining operations, these tools can significantly contribute to the efficiency and reliability of renewable energy systems. As the industry continues to evolve, organizations that leverage AI speech technology will be well-positioned to lead the way in sustainable energy management.
Keyword: AI speech tools for maintenance