Machine Learning Enhancing Return to Play Decisions in Sports
Topic: AI Sports Tools
Industry: Sports Medicine and Rehabilitation
Discover how machine learning transforms return-to-play decisions in sports medicine by balancing athlete performance and safety through AI-driven insights and tools.
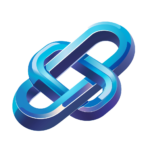
Machine Learning in Return-to-Play Decisions: Balancing Performance and Safety
In the realm of sports medicine and rehabilitation, the integration of artificial intelligence (AI) and machine learning (ML) is revolutionizing how athletes recover and return to play. The decision-making process surrounding an athlete’s readiness to resume competitive activities is critical, as it directly impacts both performance and safety. By leveraging AI-driven tools, medical professionals can make more informed decisions that balance these two essential aspects.
The Role of Machine Learning in Sports Medicine
Machine learning algorithms analyze vast amounts of data, identifying patterns and correlations that may not be immediately apparent to human practitioners. This capability is particularly beneficial in predicting an athlete’s readiness to return to play after an injury. By incorporating data from various sources, such as physical assessments, biomechanical analysis, and even psychological evaluations, AI can provide a comprehensive view of an athlete’s condition.
Key Areas of Implementation
Several key areas illustrate how machine learning can be effectively implemented in return-to-play decisions:
- Injury Prediction: AI tools can analyze historical injury data to identify risk factors and predict potential injuries before they occur. For example, systems like Catapult Sports utilize wearable technology to monitor athletes’ movements and stress levels, allowing coaches to make proactive adjustments.
- Performance Monitoring: Continuous performance tracking through AI can help determine when an athlete is ready to return. Tools like Kitman Labs use data analytics to provide insights into an athlete’s physical readiness and fatigue levels, ensuring that they are not only physically capable but also psychologically prepared for competition.
- Rehabilitation Progress: Machine learning can track rehabilitation progress through regular assessments and feedback loops. Products like Physitrack utilize AI to tailor rehabilitation programs to individual needs, adjusting exercises based on real-time performance metrics.
Examples of AI-Driven Products
Several innovative AI-driven products are at the forefront of this transformation in sports medicine:
1. Zebra Medical Vision
Zebra Medical Vision offers an AI platform that analyzes medical imaging to assist in diagnosing sports injuries. By providing accurate assessments, practitioners can make more informed decisions regarding an athlete’s readiness to return to play.
2. Kinexon
Kinexon provides a real-time tracking system that monitors player movements and workload during training and games. This data can be crucial for understanding an athlete’s physical state and ensuring they are not pushed beyond safe limits.
3. DARI Motion
DARI Motion employs AI to analyze biomechanics through motion capture technology. By assessing an athlete’s movement patterns, DARI can identify abnormalities that may indicate a higher risk of re-injury, allowing for more tailored rehabilitation strategies.
Challenges and Considerations
While the benefits of integrating AI in return-to-play decisions are significant, there are challenges that must be addressed:
- Data Privacy: The collection and analysis of personal health data raise concerns regarding privacy and consent. It is essential for organizations to establish clear protocols to protect athletes’ information.
- Integration with Existing Practices: For AI tools to be effective, they must be seamlessly integrated into existing medical practices. This requires training for medical staff and a willingness to adapt traditional methods.
- Reliability of Algorithms: The accuracy of AI-driven recommendations depends on the quality of the data and the algorithms used. Continuous validation and refinement of these systems are necessary to ensure their effectiveness.
Conclusion
The integration of machine learning in return-to-play decisions represents a significant advancement in sports medicine and rehabilitation. By harnessing the power of AI, medical professionals can better balance the dual imperatives of performance and safety. As technology continues to evolve, the potential for AI-driven tools to enhance athlete care will only grow, paving the way for safer and more effective return-to-play protocols.
Keyword: machine learning return to play