Future of Load Forecasting with AI and Smart Grid Technologies
Topic: AI Weather Tools
Industry: Energy and Utilities
Discover how AI weather tools and smart grid technologies are transforming load forecasting for utilities enhancing accuracy efficiency and sustainability in energy management
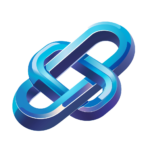
The Future of Load Forecasting: AI Weather Tools and Smart Grid Technologies
Introduction to Load Forecasting
Load forecasting is a critical aspect of energy management, enabling utilities to predict electricity demand and optimize supply accordingly. With the advent of advanced technologies, particularly artificial intelligence (AI), the landscape of load forecasting is undergoing a significant transformation. AI weather tools and smart grid technologies are set to revolutionize how energy providers approach demand forecasting, ensuring efficiency and reliability in service delivery.
The Role of AI in Load Forecasting
Artificial intelligence enhances load forecasting by analyzing vast datasets, identifying patterns, and making predictions with greater accuracy than traditional methods. By integrating AI with weather data, utilities can anticipate changes in energy demand driven by environmental factors, such as temperature fluctuations and severe weather events.
Key Benefits of AI-Driven Load Forecasting
- Increased Accuracy: AI algorithms can process historical data and real-time weather information to produce more precise forecasts.
- Enhanced Decision-Making: Utilities can make informed decisions about energy generation and distribution, minimizing waste and optimizing resources.
- Cost Efficiency: Accurate load forecasting reduces operational costs by aligning supply with actual demand, preventing overproduction and underutilization of resources.
AI Weather Tools for Energy Utilities
Several AI-driven tools and products are currently available that can significantly enhance load forecasting capabilities:
1. IBM Watson for Weather
IBM Watson leverages AI to deliver hyper-local weather forecasts. By integrating this tool with utility operations, companies can improve their load forecasting accuracy, particularly during extreme weather conditions. The platform analyzes historical weather data and real-time inputs to predict energy demand spikes due to temperature changes or storms.
2. Siemens’ MindSphere
Siemens’ MindSphere is an open IoT operating system that connects physical infrastructure to the digital world. It utilizes AI analytics to optimize energy usage and load forecasting by providing insights based on weather conditions and consumption patterns. Utilities can use this tool to anticipate demand and adjust their generation strategies accordingly.
3. Enel’s Advanced Weather Forecasting System
Enel has developed an advanced weather forecasting system that employs machine learning algorithms to analyze meteorological data. This system helps predict energy demand more accurately, allowing for better alignment of renewable energy generation with consumption patterns. By using this technology, Enel can enhance grid stability and reduce reliance on fossil fuels.
Smart Grid Technologies: The Perfect Companion
Smart grid technologies complement AI weather tools by facilitating real-time data exchange and automated decision-making processes. These technologies enable utilities to respond swiftly to changes in demand and supply, ensuring a more resilient energy infrastructure.
Integration of Smart Grids and AI
Integrating AI weather tools with smart grid technologies allows for a seamless flow of information between energy producers and consumers. For example, smart meters can provide real-time consumption data, which, when analyzed with AI algorithms, can predict future demand and optimize energy distribution accordingly.
Case Study: Pacific Gas and Electric (PG&E)
Pacific Gas and Electric has successfully implemented AI-driven load forecasting tools in conjunction with smart grid technologies. By utilizing machine learning algorithms that analyze weather patterns and consumption data, PG&E has improved its demand forecasting accuracy, leading to enhanced operational efficiency and reduced costs.
Conclusion
The future of load forecasting lies in the synergy between AI weather tools and smart grid technologies. As energy consumption patterns continue to evolve, utilities must adopt innovative solutions to meet demand reliably and sustainably. By leveraging AI-driven products and smart grid integration, energy providers can not only enhance their forecasting capabilities but also contribute to a more resilient and efficient energy ecosystem.
Keyword: AI load forecasting technologies