Machine Learning Enhances Renewable Energy Output Forecasting
Topic: AI Weather Tools
Industry: Energy and Utilities
Discover how machine learning enhances renewable energy forecasting accuracy optimizing operations and supporting a sustainable energy future
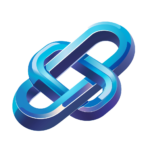
The Role of Machine Learning in Predicting Renewable Energy Output
Understanding the Importance of Accurate Renewable Energy Forecasting
As the global demand for renewable energy sources continues to rise, the need for precise forecasting of energy output has become increasingly vital. Accurate predictions enable energy producers to optimize their operations, manage supply and demand effectively, and minimize costs. In this context, machine learning (ML) has emerged as a powerful tool for enhancing the accuracy of renewable energy output predictions.
Machine Learning: A Game Changer for Energy Forecasting
Machine learning algorithms analyze vast amounts of historical and real-time data to identify patterns and trends that traditional forecasting methods may overlook. By leveraging these advanced analytical capabilities, energy companies can make informed decisions that enhance grid stability and efficiency.
How Machine Learning Works in Energy Forecasting
Machine learning models utilize various data inputs, including weather conditions, historical energy production data, and geographical information. These models are trained on this data to recognize correlations between weather variables and energy output, allowing for more accurate predictions. Common ML techniques employed in this sector include:
- Regression Analysis: Used to predict continuous output values based on input features.
- Time Series Analysis: Helps in understanding and forecasting energy production trends over time.
- Neural Networks: Particularly useful for complex pattern recognition in large datasets.
AI Weather Tools for Energy and Utilities
Several AI-driven products and tools are available that harness machine learning to enhance renewable energy forecasting. Below are some notable examples:
1. IBM Watson for Weather
IBM Watson for Weather utilizes advanced machine learning algorithms to provide hyper-local weather forecasts. By integrating weather data with energy consumption patterns, this tool helps utilities optimize energy generation and distribution. The ability to predict weather patterns with high accuracy enables energy companies to adjust their operations proactively.
2. Enel’s Energy Forecasting Platform
Enel, a global leader in renewable energy, has developed an energy forecasting platform that employs machine learning to predict solar and wind energy output. This platform uses a combination of satellite data, weather forecasts, and historical generation data to enhance the accuracy of its predictions, allowing for better planning and integration of renewable sources into the grid.
3. Google’s DeepMind AI
Google’s DeepMind has been applied in the energy sector to optimize energy usage in data centers and improve the forecasting of renewable energy production. By analyzing weather data and energy output, DeepMind’s AI models can predict energy generation from wind and solar sources with remarkable precision, leading to significant cost savings and efficiency improvements.
Implementing AI in Energy Operations
To effectively implement machine learning in energy forecasting, companies must consider several key steps:
1. Data Collection and Management
Gathering high-quality, relevant data is crucial. This includes historical energy production data, weather patterns, and geographical information. Establishing robust data management practices ensures that the data used for training machine learning models is accurate and up-to-date.
2. Model Development and Training
Once the data is collected, companies can develop machine learning models tailored to their specific forecasting needs. This involves selecting appropriate algorithms, training the models using historical data, and validating their performance through testing.
3. Integration with Existing Systems
For machine learning models to be effective, they must be integrated with existing energy management systems. This allows for real-time data analysis and enables utilities to respond quickly to changing conditions.
Conclusion
The integration of machine learning in predicting renewable energy output represents a significant advancement for the energy sector. By employing AI weather tools, energy producers can enhance forecasting accuracy, optimize operations, and ultimately contribute to a more sustainable energy future. As technology continues to evolve, the potential for machine learning in energy forecasting will only expand, paving the way for innovative solutions that meet the growing demands of the renewable energy landscape.
Keyword: machine learning renewable energy forecasting