AI Load Balancing for Grid Stability with Distributed Energy Resources
Topic: AI Website Tools
Industry: Energy and Utilities
Discover how AI-enabled load balancing enhances grid stability amidst the rise of distributed energy resources for a resilient and efficient energy future
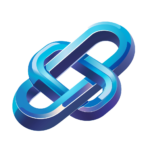
AI-Enabled Load Balancing: Ensuring Grid Stability in the Age of Distributed Energy Resources
Understanding the Need for Load Balancing
As the energy landscape evolves, the integration of Distributed Energy Resources (DERs) such as solar panels, wind turbines, and energy storage systems has become increasingly prevalent. While these resources offer numerous benefits, they also introduce complexities that can destabilize the power grid. Effective load balancing is essential to maintain grid stability, ensuring that supply meets demand in real-time. This is where artificial intelligence (AI) emerges as a transformative solution.
The Role of AI in Load Balancing
AI can enhance load balancing by analyzing vast amounts of data, predicting energy consumption patterns, and optimizing the distribution of energy resources. By leveraging machine learning algorithms, utilities can forecast demand fluctuations and adjust supply accordingly. This proactive approach not only enhances grid reliability but also supports the integration of renewable energy sources.
Key AI Techniques for Load Balancing
Several AI techniques can be employed to improve load balancing in the energy sector:
- Predictive Analytics: Utilizing historical data to predict future energy consumption trends.
- Real-Time Data Processing: Analyzing data from smart meters and IoT devices to make instantaneous decisions.
- Optimization Algorithms: Implementing algorithms that determine the most efficient distribution of energy resources.
AI-Driven Tools and Products
There are several AI-driven tools and platforms that can assist utilities in implementing effective load balancing strategies:
1. Siemens Spectrum Power
Siemens Spectrum Power is a comprehensive energy management system that utilizes AI to enhance grid operations. By integrating predictive analytics, it enables utilities to anticipate demand and optimize resource allocation. This tool is particularly beneficial for managing the complexities introduced by DERs.
2. IBM Watson IoT
IBM Watson IoT offers advanced analytics capabilities that allow energy providers to monitor and manage distributed energy resources effectively. Its machine learning algorithms can identify patterns in energy usage, enabling utilities to make data-driven decisions for load balancing.
3. GE Digital’s Digital Wind Farm
GE Digital’s Digital Wind Farm utilizes AI to optimize the performance of wind energy systems. By analyzing real-time data from wind turbines, it can predict energy output and adjust load balancing strategies accordingly, ensuring that energy supply aligns with demand.
Challenges and Considerations
While the integration of AI into load balancing presents numerous advantages, there are challenges that utilities must address:
- Data Security: Ensuring the security of sensitive data collected from various sources is paramount.
- Integration with Legacy Systems: Many utilities still rely on outdated infrastructure, making it difficult to implement new AI technologies.
- Regulatory Compliance: Adhering to regulations while adopting AI solutions can be complex and requires careful planning.
Conclusion
As the energy sector continues to transition towards a more decentralized model, AI-enabled load balancing will play a crucial role in ensuring grid stability. By leveraging advanced analytics and optimization tools, utilities can effectively manage the challenges posed by distributed energy resources. The future of energy management lies in the successful integration of AI technologies, paving the way for a more resilient and efficient grid.
Keyword: AI load balancing for energy management