Addressing Algorithmic Bias in AI Academic Recruitment
Topic: AI Recruitment Tools
Industry: Education
Explore ethical considerations in AI-powered academic recruitment and learn how to address algorithmic bias for fair and inclusive hiring practices in education.
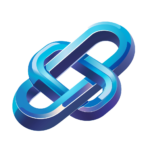
Ethical Considerations: Addressing Algorithmic Bias in AI-Powered Academic Recruitment
The Rise of AI in Academic Recruitment
In recent years, the integration of artificial intelligence (AI) in academic recruitment processes has transformed how educational institutions attract, evaluate, and select candidates. AI-powered recruitment tools offer the potential for increased efficiency, reduced bias, and enhanced candidate experience. However, as these technologies become more prevalent, it is imperative to address the ethical considerations surrounding algorithmic bias that may inadvertently influence hiring decisions.
Understanding Algorithmic Bias
Algorithmic bias occurs when an AI system produces results that are systematically prejudiced due to flawed assumptions in the machine learning process. This can manifest in various forms, including gender, racial, or socioeconomic biases, which may lead to the exclusion of qualified candidates from diverse backgrounds. It is crucial for educational institutions to recognize and mitigate these biases to ensure fair and equitable recruitment practices.
Impacts of Bias in Recruitment
The repercussions of algorithmic bias in academic recruitment can be significant. For instance, biased algorithms may favor candidates who fit a particular profile, thereby perpetuating existing disparities in representation among faculty and staff. This not only undermines the principles of diversity and inclusion but can also affect the institution’s reputation and ability to attract top talent.
Implementing AI Responsibly
To harness the benefits of AI while minimizing the risks of bias, educational institutions must adopt a responsible approach to the implementation of AI-powered recruitment tools. This involves several key strategies:
1. Data Auditing and Cleaning
Before deploying AI recruitment tools, institutions should conduct thorough audits of the data used to train algorithms. This includes examining historical hiring data for potential biases and ensuring that the datasets reflect a diverse range of candidates. Cleaning the data to remove any biased or irrelevant information is essential for developing fair algorithms.
2. Transparent Algorithms
Transparency in algorithm design is crucial for accountability. Educational institutions should seek AI tools that offer insights into how decisions are made. For example, tools like HireVue provide detailed explanations of their AI-driven assessment processes, allowing institutions to understand the rationale behind candidate evaluations.
3. Continuous Monitoring and Feedback
AI systems should not be viewed as set-and-forget solutions. Continuous monitoring of AI-driven recruitment processes is essential to identify and rectify any emerging biases. Feedback loops involving diverse stakeholders can help institutions refine their algorithms and ensure they align with ethical standards.
Examples of AI-Powered Recruitment Tools
Several AI-driven products are available to assist educational institutions in their recruitment efforts while addressing algorithmic bias:
1. LinkedIn Talent Insights
This tool leverages AI to analyze talent pools and provide insights into candidate demographics, skill sets, and trends. By utilizing this data, institutions can make informed decisions that prioritize diversity and inclusion.
2. Pymetrics
Pymetrics uses neuroscience-based games and AI to assess candidates’ cognitive and emotional traits. The platform aims to reduce bias by focusing on an individual’s potential rather than their background, helping institutions identify diverse talent.
3. Eightfold.ai
Eightfold.ai offers a talent intelligence platform that uses AI to match candidates with job opportunities based on their skills and experiences. The platform emphasizes equitable hiring practices by actively working to eliminate bias in its matching algorithms.
Conclusion
As educational institutions increasingly adopt AI-powered recruitment tools, it is essential to address the ethical considerations surrounding algorithmic bias. By implementing responsible practices, such as data auditing, transparency, and continuous monitoring, institutions can leverage the benefits of AI while fostering diversity and inclusion within their academic communities. The future of recruitment in education lies not only in technological advancement but also in our commitment to ethical practices that uphold fairness and equity.
Keyword: ethical AI in academic recruitment