AI A/B Testing Strategies for Optimizing Marketing Campaigns
Topic: AI Business Tools
Industry: Marketing and Advertising
Discover how AI-powered A/B testing optimizes marketing campaigns using machine learning for faster insights and improved performance in your strategies.
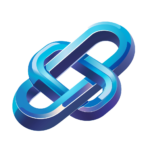
AI-Powered A/B Testing: Optimizing Campaigns with Machine Learning
Understanding A/B Testing in Marketing
A/B testing, also known as split testing, is a fundamental practice in marketing that involves comparing two versions of a campaign to determine which one performs better. Traditionally, this process required marketers to rely on manual analysis and intuition. However, with the advent of artificial intelligence (AI), A/B testing has evolved into a more sophisticated and efficient process. AI-driven tools can analyze vast amounts of data quickly, providing insights that lead to more informed decision-making.
The Role of AI in A/B Testing
Artificial intelligence enhances A/B testing by automating data analysis, predicting outcomes, and optimizing campaigns in real-time. By leveraging machine learning algorithms, marketers can identify patterns and trends that would be challenging to detect manually. This capability allows for a more dynamic approach to testing, where campaigns can be adjusted on the fly based on real-time performance data.
Key Benefits of AI-Powered A/B Testing
- Speed: AI can process data at an unprecedented rate, allowing marketers to run tests and receive results much faster than traditional methods.
- Precision: Machine learning algorithms can deliver more accurate predictions about campaign performance, reducing the likelihood of human error.
- Scalability: AI tools can handle multiple tests simultaneously, enabling marketers to scale their efforts without sacrificing quality.
- Continuous Improvement: With AI, marketers can implement a continuous feedback loop that refines campaigns over time, rather than relying on one-off tests.
Implementing AI in A/B Testing
To effectively implement AI in A/B testing, businesses can utilize various tools and platforms designed to integrate machine learning into their marketing strategies. Here are some notable examples:
1. Google Optimize
Google Optimize is a powerful tool that allows marketers to run A/B tests and multivariate tests seamlessly. Its integration with Google Analytics enables users to leverage AI-driven insights to optimize their campaigns. By analyzing user behavior and demographics, Google Optimize helps marketers tailor their content to specific audience segments.
2. Optimizely
Optimizely is another leading platform that utilizes machine learning to enhance A/B testing. It provides advanced targeting capabilities and real-time analytics, allowing businesses to make data-driven decisions quickly. Optimizely’s AI features can automatically recommend variations based on user interactions, streamlining the testing process.
3. Adobe Target
Adobe Target employs AI to deliver personalized experiences through A/B testing and automated targeting. Its machine learning algorithms analyze user data to predict which variations will yield the best results. This tool is particularly beneficial for businesses looking to enhance customer engagement and conversion rates.
4. VWO (Visual Website Optimizer)
VWO offers a comprehensive suite of testing tools, including A/B testing, multivariate testing, and split URL testing. Its AI capabilities help marketers identify the most impactful changes to their campaigns by analyzing user behavior and engagement metrics. VWO’s predictive analytics feature allows marketers to forecast the potential success of different variations before launching a full-scale test.
Best Practices for AI-Powered A/B Testing
To maximize the benefits of AI in A/B testing, marketers should consider the following best practices:
- Define Clear Objectives: Establish specific goals for each test to ensure that the results are actionable and aligned with overall business objectives.
- Segment Your Audience: Utilize AI tools to segment your audience effectively, allowing for more personalized and relevant testing.
- Run Tests Simultaneously: Conduct A/B tests concurrently to reduce the impact of external variables and gain more reliable insights.
- Analyze Results Thoroughly: Leverage AI analytics to dive deep into the data, identifying not just what worked, but why it worked.
Conclusion
AI-powered A/B testing represents a significant advancement in the realm of marketing and advertising. By implementing machine learning tools, businesses can optimize their campaigns more effectively, driving better results and enhancing customer engagement. As AI technology continues to evolve, the potential for more sophisticated and impactful A/B testing will only expand, making it an essential component of any modern marketing strategy.
Keyword: AI powered A/B testing