AI Analytics in Healthcare Interoperability for 2025
Topic: AI Analytics Tools
Industry: Healthcare
Discover how AI analytics is transforming healthcare interoperability in 2025 by breaking down data silos to enhance patient care and collaboration
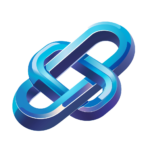
AI Analytics for Healthcare Interoperability: Breaking Down Data Silos in 2025
Understanding the Challenge of Data Silos in Healthcare
In the rapidly evolving landscape of healthcare, the ability to share and access data seamlessly across different systems is paramount. Data silos, where information is trapped within individual departments or organizations, pose significant challenges to achieving interoperability. These silos hinder collaboration, reduce efficiency, and ultimately impact patient care. In 2025, as healthcare continues to embrace digital transformation, artificial intelligence (AI) analytics tools are set to play a pivotal role in breaking down these barriers.
The Role of AI in Healthcare Interoperability
Artificial intelligence has the potential to revolutionize how healthcare organizations manage and share data. By leveraging advanced algorithms and machine learning, AI can analyze vast amounts of data from disparate sources, providing insights that were previously unattainable. This capability not only enhances decision-making but also fosters a more integrated approach to patient care.
Key AI Analytics Tools for Interoperability
Several AI-driven products are emerging as leaders in the quest for interoperability in healthcare. Below are some notable examples:
1. IBM Watson Health
IBM Watson Health utilizes AI to analyze healthcare data from various sources, including electronic health records (EHRs), clinical trials, and patient-reported outcomes. By employing natural language processing and machine learning, Watson can identify patterns and correlations that may inform treatment decisions and improve patient outcomes.
2. Google Cloud Healthcare API
The Google Cloud Healthcare API provides a robust framework for managing and sharing healthcare data across different platforms. By integrating AI capabilities, organizations can enhance their data analytics processes, enabling real-time insights and facilitating better care coordination among providers.
3. Microsoft Azure Health Bot
Microsoft’s Azure Health Bot leverages AI to streamline patient interactions and data collection. This tool can assist healthcare providers in triaging patients and gathering relevant health information, which can then be integrated into EHR systems, thereby reducing data silos and enhancing interoperability.
Implementing AI Analytics in Healthcare
Implementing AI analytics tools requires a strategic approach. Here are some steps healthcare organizations can take to effectively integrate these technologies:
1. Assess Current Data Infrastructure
Before implementing AI solutions, organizations must evaluate their existing data infrastructure. Understanding the current state of data collection, storage, and sharing practices will help identify gaps and areas for improvement.
2. Choose the Right AI Tools
Selecting the appropriate AI analytics tools is crucial. Organizations should consider factors such as scalability, compatibility with existing systems, and the specific needs of their patient population when choosing a solution.
3. Foster a Culture of Collaboration
Breaking down data silos requires collaboration among various stakeholders, including clinicians, IT professionals, and administrative staff. Fostering a culture of teamwork and open communication will facilitate the successful implementation of AI analytics tools.
4. Ensure Compliance and Security
As healthcare organizations adopt AI technologies, ensuring compliance with regulations such as HIPAA is essential. Organizations must implement robust data security measures to protect sensitive patient information while enabling data sharing.
The Future of AI in Healthcare Interoperability
As we look toward 2025, the integration of AI analytics tools in healthcare will continue to advance, driving improvements in interoperability and patient care. By breaking down data silos, healthcare organizations can create a more connected ecosystem that enhances collaboration, improves outcomes, and ultimately transforms the patient experience.
Conclusion
AI analytics tools are not just a trend; they represent a fundamental shift in how healthcare organizations approach data management and interoperability. By embracing these technologies, healthcare providers can overcome the challenges posed by data silos and create a more cohesive and efficient healthcare system.
Keyword: AI analytics healthcare interoperability