AI Analytics in Lending Streamlining Credit Decisions and Underwriting
Topic: AI Analytics Tools
Industry: Finance and Banking
Discover how AI analytics transforms lending by streamlining credit decisions and underwriting for improved efficiency and customer satisfaction in financial services
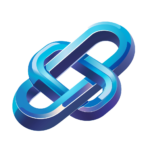
AI Analytics in Lending: Streamlining Credit Decisions and Underwriting
The Role of AI in Financial Services
Artificial Intelligence (AI) has revolutionized numerous industries, and the finance sector is no exception. In lending, AI analytics tools are transforming how financial institutions assess creditworthiness, manage risk, and streamline underwriting processes. By leveraging vast amounts of data, AI can facilitate faster, more accurate decision-making, ultimately enhancing customer experience and operational efficiency.
Understanding AI Analytics Tools
AI analytics tools encompass a range of technologies designed to analyze data, predict outcomes, and automate processes. In the context of lending, these tools can assess borrower profiles, evaluate credit risk, and optimize underwriting workflows. The implementation of AI analytics can reduce human error, eliminate bias, and provide a more comprehensive view of a borrower’s financial health.
Key Benefits of AI Analytics in Lending
- Enhanced Decision-Making: AI algorithms can analyze historical data and identify patterns that traditional methods may overlook, leading to more informed credit decisions.
- Improved Efficiency: Automating data analysis and underwriting processes reduces the time required to process loan applications, allowing lenders to serve more customers effectively.
- Risk Mitigation: AI tools can assess a wider range of variables, helping lenders identify potential risks and avoid defaults.
- Personalized Offerings: By analyzing customer data, lenders can tailor loan products to meet individual borrower needs, enhancing customer satisfaction.
Implementing AI Analytics in Lending
To effectively integrate AI analytics into lending practices, financial institutions should consider the following steps:
1. Data Collection and Integration
Gathering relevant data from various sources is crucial. This includes credit reports, transaction histories, and alternative data sources such as social media activity and payment histories. Integrating this data into a centralized system allows for comprehensive analysis.
2. Selecting the Right AI Tools
Choosing the appropriate AI analytics tools is vital for successful implementation. Several AI-driven products stand out in the lending landscape:
Credit Scoring Solutions
Tools like FICO Score and Experian Boost utilize AI to enhance traditional credit scoring models. These solutions analyze alternative data to provide a more accurate assessment of a borrower’s creditworthiness.
Automated Underwriting Systems
Systems such as Zest AI and Upstart employ machine learning algorithms to automate the underwriting process. These platforms evaluate a broader range of data points, enabling faster and more precise loan approvals.
Fraud Detection Tools
AI-driven fraud detection tools like Kount and Feedzai analyze transaction patterns in real-time to identify suspicious activities, helping lenders mitigate the risk of fraud.
3. Continuous Monitoring and Improvement
Once AI analytics tools are in place, continuous monitoring is essential. Financial institutions should regularly assess the performance of their AI systems, making adjustments as necessary to improve accuracy and effectiveness.
Conclusion
The integration of AI analytics in lending is not just a trend; it is a necessary evolution in the financial services industry. By implementing AI-driven tools, lenders can streamline credit decisions and underwriting processes, ultimately enhancing their ability to serve customers while minimizing risk. As AI technology continues to advance, its role in lending will only become more critical, paving the way for a more efficient and equitable financial landscape.
Keyword: AI analytics in lending