AI Analytics Transforming Agricultural Supply Chains from Farm to Table
Topic: AI Analytics Tools
Industry: Agriculture
Discover how AI analytics optimizes agricultural supply chains from farm to table enhancing efficiency reducing waste and improving decision-making processes
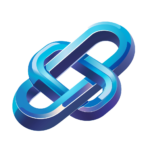
AI Analytics for Optimizing Supply Chains in Agriculture: From Farm to Table
Understanding the Role of AI in Agriculture
Artificial intelligence (AI) is transforming various sectors, and agriculture is no exception. The integration of AI analytics tools into agricultural supply chains enhances efficiency, reduces waste, and improves decision-making processes. By leveraging data-driven insights, farmers and agribusinesses can optimize their operations from cultivation to distribution, ensuring that products reach consumers in a timely and cost-effective manner.
The Importance of Supply Chain Optimization
Effective supply chain management is crucial in agriculture, where products are perishable and demand can fluctuate. Optimizing supply chains not only minimizes losses but also maximizes profitability. AI analytics tools can analyze vast amounts of data to identify patterns, forecast demand, and streamline logistics, ultimately leading to a more resilient and responsive agricultural supply chain.
Key Areas Where AI Analytics Can Be Implemented
AI analytics can be applied at various stages of the agricultural supply chain:
1. Crop Management
AI tools can assist farmers in monitoring crop health and predicting yields. For instance, platforms like IBM Watson Decision Platform for Agriculture utilize AI algorithms to analyze data from sensors, weather forecasts, and soil conditions to provide actionable insights tailored to specific crops.
2. Inventory Management
Efficient inventory management is essential for minimizing waste. AI-driven tools such as AgriWebb offer real-time visibility into stock levels and automate reordering processes based on predictive analytics. This ensures that farmers have the right amount of supplies without overstocking.
3. Demand Forecasting
Understanding market demand is vital for planning production cycles. AI analytics platforms like Farmshelf leverage historical sales data and market trends to forecast demand accurately, enabling farmers to adjust their planting and harvesting schedules accordingly.
4. Logistics and Distribution
AI can optimize transportation routes and schedules, reducing delivery times and costs. Tools such as FarmLogs utilize machine learning algorithms to analyze traffic patterns and weather conditions, helping logistics managers make informed decisions about the best routes for transporting goods.
Case Studies: Successful Implementation of AI in Agriculture
Several agricultural enterprises have successfully integrated AI analytics into their supply chains, yielding impressive results:
Case Study 1: John Deere
John Deere has embraced AI through its precision agriculture solutions. By utilizing AI-driven analytics, the company provides farmers with insights on soil health and crop performance, enabling them to make data-informed decisions that enhance productivity.
Case Study 2: Cargill
Cargill, a global leader in food production, employs AI analytics to optimize its supply chains. By analyzing data from various sources, including weather patterns and market trends, Cargill can anticipate changes in demand and adjust its operations accordingly, thereby reducing waste and improving efficiency.
Challenges and Considerations
While the benefits of AI analytics in agriculture are significant, there are challenges to consider. Data privacy, the need for skilled personnel, and the initial investment in technology can pose obstacles for some organizations. However, the long-term advantages of improved efficiency and reduced costs often outweigh these challenges.
Conclusion
As the agricultural industry continues to evolve, the integration of AI analytics tools will play a pivotal role in optimizing supply chains from farm to table. By harnessing the power of AI, farmers and agribusinesses can enhance their operations, reduce waste, and ultimately deliver fresher, more sustainable products to consumers. The future of agriculture is undoubtedly data-driven, and those who embrace AI analytics will be well-positioned to thrive in this competitive landscape.
Keyword: AI analytics in agricultural supply chains