AI Audio Analytics for Enhanced Audience Engagement Insights
Topic: AI Audio Tools
Industry: Media and Broadcasting
Discover how AI audio analytics enhances audience engagement through machine learning insights for media and broadcasting organizations. Drive growth and retention today.
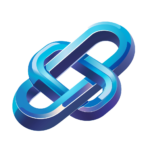
AI Audio Analytics: Using Machine Learning to Understand Audience Engagement
Understanding the Importance of Audience Engagement
In the rapidly evolving landscape of media and broadcasting, understanding audience engagement has become paramount. With the proliferation of content across various platforms, it is essential for broadcasters and media companies to gain insights into how their audiences interact with audio content. This is where AI audio analytics, powered by machine learning, comes into play.
What is AI Audio Analytics?
AI audio analytics refers to the application of artificial intelligence techniques to analyze audio data. By leveraging machine learning algorithms, media organizations can extract valuable insights from audio content, enabling them to understand listener behavior, preferences, and engagement levels. This analysis can lead to more informed content creation and marketing strategies, ultimately driving audience retention and growth.
Implementing AI in Audio Analytics
Implementing AI in audio analytics involves several key steps, including data collection, preprocessing, model training, and analysis. Here’s a closer look at how this process unfolds:
1. Data Collection
The first step in AI audio analytics is the collection of audio data. This can include recordings from podcasts, radio shows, and other audio content. The data can be sourced from various platforms, including streaming services and social media channels.
2. Preprocessing
Once the audio data is collected, it must be preprocessed to ensure it is suitable for analysis. This may involve noise reduction, normalization, and segmentation of audio files to isolate specific segments for analysis.
3. Model Training
Machine learning models are then trained on the preprocessed audio data. These models can be designed to recognize patterns in listener engagement, such as peak listening times, drop-off points, and sentiment analysis. Techniques such as natural language processing (NLP) can also be employed to analyze spoken content for thematic trends.
4. Analysis and Insights
After the models are trained, they can be used to analyze new audio content in real-time. The insights generated can help media organizations understand what resonates with their audience, allowing them to tailor content accordingly.
Examples of AI-Driven Tools in Audio Analytics
Several tools and products are currently available that leverage AI for audio analytics in the media and broadcasting industry. Here are a few noteworthy examples:
1. Descript
Descript is an innovative audio editing tool that utilizes AI to transcribe audio files into text. This allows content creators to easily edit their audio by editing the text. Additionally, Descript offers features such as speaker identification and engagement tracking, providing valuable insights into audience interaction.
2. Auphonic
Auphonic is an AI-driven audio processing tool that enhances audio quality by automatically adjusting levels, reducing noise, and applying various audio effects. It also provides analytics on listener engagement, helping creators understand how their audience interacts with their content.
3. Podtrac
Podtrac offers analytics specifically designed for podcasters. Their service provides detailed metrics on audience engagement, including listener demographics and behavior patterns. By utilizing AI algorithms, Podtrac helps podcasters make data-driven decisions to improve their content and marketing strategies.
4. Sonosuite
Sonosuite is a comprehensive audio distribution platform that includes AI-powered analytics tools. It provides insights into listener behavior across multiple platforms, helping broadcasters optimize their content for maximum reach and engagement.
The Future of AI Audio Analytics
As the media and broadcasting industry continues to evolve, the role of AI audio analytics will only become more significant. Organizations that embrace these technologies will be better positioned to understand their audiences and create content that resonates. By leveraging machine learning and AI-driven tools, media companies can enhance their decision-making processes, leading to increased audience engagement and loyalty.
Conclusion
In conclusion, AI audio analytics represents a transformative opportunity for media and broadcasting organizations. By implementing machine learning techniques and utilizing advanced tools, businesses can gain a deeper understanding of their audience engagement, paving the way for more effective content strategies and enhanced viewer satisfaction. As technology continues to advance, those who harness the power of AI will undoubtedly lead the way in the competitive landscape of media and broadcasting.
Keyword: AI audio analytics for engagement