AI Driven Anomaly Detection for Utility Control Room Safety
Topic: AI Security Tools
Industry: Energy and Utilities
Discover how AI-driven anomaly detection enhances reliability and safety in utility control rooms by identifying irregularities and optimizing operations.
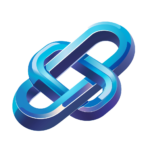
AI-Driven Anomaly Detection: Safeguarding Utility Control Rooms
The Importance of Anomaly Detection in Utility Control Rooms
In the energy and utilities sector, control rooms serve as the nerve center for monitoring and managing critical infrastructure. Any disruption or anomaly can lead to significant operational inefficiencies, safety hazards, and financial losses. Therefore, implementing robust anomaly detection mechanisms is essential for maintaining high operational standards and ensuring the reliability of services.Understanding AI-Driven Anomaly Detection
Artificial Intelligence (AI) has revolutionized the approach to anomaly detection by enabling systems to learn from historical data, identify patterns, and flag irregularities in real-time. Unlike traditional methods that rely heavily on predefined thresholds, AI-driven solutions utilize machine learning algorithms to adapt and improve over time, providing a more dynamic and responsive approach to anomaly detection.How AI Can Be Implemented in Utility Control Rooms
The integration of AI in utility control rooms can be accomplished through several key steps: 1. Data Collection and Integration: The first step involves gathering data from various sources, including sensors, SCADA systems, and historical databases. This data serves as the foundation for training AI models. 2. Model Training: By employing machine learning techniques, organizations can train models to recognize normal operational patterns. This training phase is crucial for enabling the system to distinguish between typical behavior and potential anomalies. 3. Real-Time Monitoring: Once trained, AI models can be deployed for real-time monitoring. They continuously analyze incoming data, providing immediate alerts when deviations from established patterns occur. 4. Feedback Loop: Implementing a feedback mechanism allows the system to learn from false positives and false negatives, refining its accuracy over time.Examples of AI-Driven Tools for Anomaly Detection
Several AI-driven tools and products are tailored for anomaly detection in the energy and utilities sector: – IBM Watson IoT: This platform leverages AI to analyze data from connected devices, enabling utilities to predict equipment failures and optimize maintenance schedules. Its anomaly detection capabilities help identify irregular patterns that could indicate underlying issues. – Siemens MindSphere: Siemens’ cloud-based IoT operating system utilizes machine learning algorithms to detect anomalies in real-time. It allows utility companies to monitor the health of their assets and respond proactively to potential problems. – GE Digital’s Predix: Designed specifically for industrial applications, Predix employs advanced analytics and machine learning to detect anomalies in operational data. By providing insights into equipment performance, it helps utilities mitigate risks and enhance operational efficiency. – Microsoft Azure Anomaly Detector: This tool offers an easy-to-use API that allows organizations to integrate anomaly detection capabilities into their existing systems. It provides real-time insights and alerts, empowering utility control rooms to respond swiftly to potential threats.Benefits of AI-Driven Anomaly Detection
The implementation of AI-driven anomaly detection systems in utility control rooms offers numerous benefits: – Enhanced Reliability: By identifying anomalies early, utilities can prevent outages and ensure a consistent supply of energy. – Cost Savings: Proactive maintenance driven by AI insights reduces the costs associated with emergency repairs and unplanned downtime. – Improved Safety: Early detection of anomalies can prevent accidents and ensure the safety of personnel and infrastructure. – Data-Driven Decision Making: AI tools provide actionable insights that empower decision-makers to optimize operations and resource allocation.Conclusion
The integration of AI-driven anomaly detection in utility control rooms represents a significant advancement in the energy and utilities sector. By harnessing the power of artificial intelligence, organizations can safeguard their operations, enhance reliability, and ultimately deliver better services to their customers. As technology continues to evolve, the potential for AI to transform the way utilities manage their infrastructure will only grow, making it an indispensable tool in the modern energy landscape.Keyword: AI anomaly detection in utilities