AI Driven Demand Forecasting for Efficient Supply Chain Planning
Topic: AI Self Improvement Tools
Industry: Logistics and Supply Chain
Discover how AI-driven demand forecasting transforms supply chain planning with enhanced accuracy and efficiency for better decision-making and reduced costs
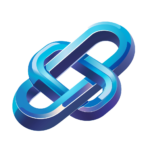
AI-Driven Demand Forecasting: The Future of Supply Chain Planning
Understanding Demand Forecasting in Supply Chain Management
Demand forecasting is a critical component of supply chain management, enabling businesses to predict customer demand and optimize inventory levels. Traditional forecasting methods often rely on historical data and basic statistical techniques, which can lead to inaccuracies and inefficiencies. As the complexity of global supply chains increases, the need for more sophisticated forecasting methods becomes paramount.
The Role of Artificial Intelligence in Demand Forecasting
Artificial intelligence (AI) is revolutionizing demand forecasting by providing advanced analytics and machine learning capabilities. AI-driven demand forecasting tools can analyze vast amounts of data, identify patterns, and generate insights that were previously unattainable through conventional methods. This results in more accurate forecasts, reduced stockouts, and improved customer satisfaction.
Key Benefits of AI-Driven Demand Forecasting
- Enhanced Accuracy: AI algorithms can process real-time data from various sources, including sales history, market trends, and external factors like weather or economic indicators, leading to more precise forecasts.
- Dynamic Adjustments: AI systems can adapt to changes in demand patterns, allowing businesses to respond swiftly to market fluctuations.
- Cost Reduction: Improved forecasting reduces excess inventory and associated holding costs, optimizing supply chain efficiency.
- Informed Decision-Making: AI-driven insights empower supply chain managers to make strategic decisions based on data rather than intuition.
Implementing AI in Demand Forecasting
To leverage AI for demand forecasting, organizations must adopt a systematic approach. This involves integrating AI tools into existing systems, ensuring data quality, and fostering a culture of data-driven decision-making. Here are some steps to consider:
1. Data Collection and Integration
Successful AI implementations begin with gathering high-quality data from various sources, including ERP systems, CRM platforms, and external market data. Integrating these data sources into a unified platform is essential for accurate analysis.
2. Selecting the Right AI Tools
There are numerous AI-driven tools available that can enhance demand forecasting capabilities. Some notable examples include:
- IBM Watson: This AI platform offers advanced analytics capabilities, enabling businesses to analyze historical data and predict future demand trends.
- Microsoft Azure Machine Learning: This cloud-based service provides tools for building, training, and deploying machine learning models tailored to specific forecasting needs.
- Forecast Pro: A specialized forecasting software that uses AI algorithms to deliver precise demand forecasts and includes features for collaboration and reporting.
- Blue Yonder: This supply chain platform leverages AI to optimize inventory management and demand planning, providing real-time insights into supply chain dynamics.
3. Continuous Improvement and Learning
AI systems thrive on continuous learning. Organizations should regularly evaluate the performance of their forecasting models and refine them based on new data and outcomes. This iterative process ensures that the AI tools remain relevant and effective in a constantly changing market environment.
Case Studies of AI-Driven Demand Forecasting
Several companies have successfully implemented AI-driven demand forecasting to enhance their supply chain operations:
Walmart
Walmart utilizes machine learning algorithms to analyze customer purchase patterns and predict demand. This approach has allowed the retail giant to optimize inventory levels and reduce stockouts, resulting in improved customer satisfaction and increased sales.
Unilever
Unilever has embraced AI to forecast demand across its extensive product range. By analyzing data from various sources, including social media and market trends, Unilever has achieved greater accuracy in its demand predictions, leading to more efficient supply chain operations.
Conclusion
AI-driven demand forecasting represents a significant advancement in supply chain planning. By implementing AI tools and fostering a culture of data-driven decision-making, organizations can enhance their forecasting accuracy, reduce costs, and improve overall supply chain efficiency. As AI technology continues to evolve, businesses that adopt these innovative tools will be well-positioned to thrive in an increasingly competitive landscape.
Keyword: AI demand forecasting tools