AI Driven Demand Forecasting for Energy Companies Efficiency
Topic: AI Business Tools
Industry: Energy and Utilities
Discover how AI-driven demand forecasting can transform energy companies by enhancing accuracy optimizing resources and improving customer satisfaction
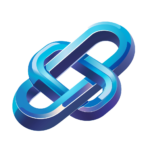
AI-Driven Demand Forecasting: A Game-Changer for Energy Companies
The Importance of Accurate Demand Forecasting
In the energy sector, accurate demand forecasting is crucial for optimizing operations, managing resources, and ensuring customer satisfaction. Traditional forecasting methods often rely on historical data and manual analysis, which can lead to inefficiencies and inaccuracies. However, with the advent of artificial intelligence (AI), energy companies can now leverage advanced algorithms to enhance their forecasting capabilities significantly.How AI Can Transform Demand Forecasting
AI-driven demand forecasting utilizes machine learning algorithms to analyze vast amounts of data, identifying patterns and trends that would be nearly impossible to detect through conventional methods. By incorporating variables such as weather patterns, economic indicators, and consumer behavior, AI can provide more accurate predictions of energy demand.Implementation of AI in Demand Forecasting
To implement AI-driven demand forecasting, energy companies can follow a systematic approach: 1. Data Collection: Gather historical consumption data, weather forecasts, economic indicators, and other relevant datasets. 2. Data Preparation: Clean and preprocess the data to ensure accuracy and consistency. This may involve handling missing values and normalizing data formats. 3. Model Selection: Choose appropriate machine learning models such as regression analysis, time series forecasting, or neural networks based on the complexity of the data and forecasting needs. 4. Training and Validation: Train the selected models using historical data and validate their performance against a separate dataset to ensure reliability. 5. Deployment: Integrate the AI model into existing forecasting systems, allowing for real-time analysis and updates. 6. Continuous Improvement: Regularly monitor the model’s performance and update it with new data to enhance accuracy over time.Examples of AI-Driven Tools and Products
Several AI-driven tools and platforms are currently available that can aid energy companies in their demand forecasting efforts: – IBM Watson: IBM’s AI platform offers predictive analytics capabilities that can be tailored for energy demand forecasting. By analyzing historical data and external factors, Watson can generate accurate demand predictions, helping companies optimize their supply chains. – Siemens MindSphere: This cloud-based IoT operating system allows energy companies to connect their devices and analyze data in real-time. By leveraging AI algorithms, MindSphere can provide insights into energy consumption patterns, enabling better demand forecasting. – Microsoft Azure Machine Learning: Azure provides a suite of machine learning tools that can be used to build, train, and deploy predictive models. Energy companies can utilize Azure’s capabilities to forecast demand based on various influencing factors, enhancing their operational efficiency. – Grid.ai: This platform specializes in simplifying the deployment of machine learning models in the energy sector. Grid.ai can help energy companies create custom models for demand forecasting, integrating seamlessly with existing data infrastructures.Benefits of AI-Driven Demand Forecasting
The adoption of AI-driven demand forecasting offers numerous benefits for energy companies: – Increased Accuracy: AI models can analyze complex datasets and provide more precise forecasts, reducing the risk of overproduction or underproduction. – Cost Efficiency: By optimizing resource allocation based on accurate demand predictions, companies can significantly reduce operational costs. – Enhanced Customer Satisfaction: With better forecasting, energy providers can ensure a reliable supply, improving customer trust and satisfaction. – Sustainability: Accurate demand forecasting contributes to more efficient energy use, supporting sustainability goals and reducing waste.Conclusion
AI-driven demand forecasting represents a transformative opportunity for energy companies looking to enhance their operational efficiency and customer service. By implementing advanced AI tools and techniques, these organizations can achieve greater accuracy in their forecasting, ultimately leading to improved decision-making and strategic planning. As the energy landscape continues to evolve, embracing AI technology will be essential for companies aiming to stay competitive and responsive to market demands.Keyword: AI demand forecasting energy companies