AI Driven Demand Forecasting Strategies for Retail Success
Topic: AI Productivity Tools
Industry: Retail and E-commerce
Discover how AI transforms demand forecasting in retail by enhancing accuracy optimizing supply chains and improving customer satisfaction for competitive advantage
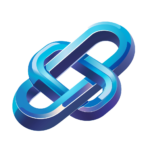
Leveraging AI for Demand Forecasting in the Retail Industry
The Importance of Demand Forecasting
In the fast-paced world of retail and e-commerce, accurate demand forecasting is crucial for maintaining inventory levels, optimizing supply chains, and enhancing customer satisfaction. Traditional forecasting methods often fall short due to their reliance on historical data and static algorithms. However, with the advent of artificial intelligence (AI), businesses can now leverage advanced analytics to predict consumer demand more accurately and effectively.
How AI Transforms Demand Forecasting
AI-driven demand forecasting utilizes machine learning algorithms to analyze vast amounts of data from various sources, including sales history, market trends, seasonality, and even social media sentiment. By identifying patterns and correlations that may not be immediately apparent, AI can provide retailers with actionable insights that lead to more informed decision-making.
Implementing AI in Demand Forecasting
To implement AI for demand forecasting, retailers should consider the following steps:
- Data Collection: Gather relevant data from multiple sources, including point-of-sale systems, customer relationship management (CRM) tools, and external market data.
- Data Cleaning: Ensure the data is clean and structured to facilitate accurate analysis. This may involve removing duplicates, correcting errors, and standardizing formats.
- Choosing the Right Tools: Select AI-driven tools that align with the specific needs of the business. This could include demand forecasting software, machine learning platforms, or analytics tools.
- Model Training: Train machine learning models on historical data to recognize patterns and make predictions about future demand.
- Continuous Monitoring: Regularly monitor the performance of AI models and adjust them as needed to improve accuracy and adapt to changing market conditions.
Examples of AI-Driven Tools for Demand Forecasting
Several AI-driven tools and platforms have emerged in recent years, offering retailers powerful capabilities for demand forecasting:
1. IBM Watson Studio
IBM Watson Studio provides a robust environment for data scientists to build, train, and deploy machine learning models. Retailers can use this tool to analyze historical sales data and external factors, allowing them to generate accurate demand forecasts.
2. Microsoft Azure Machine Learning
Microsoft Azure Machine Learning offers a comprehensive suite of tools for building and deploying machine learning models. Retailers can leverage its capabilities to analyze customer behavior and predict future demand based on real-time data.
3. Blue Yonder
Blue Yonder specializes in supply chain optimization and demand forecasting. Its AI-driven platform uses advanced algorithms to provide retailers with precise demand predictions, helping them manage inventory more effectively and reduce stockouts.
4. Forecast Pro
Forecast Pro is a user-friendly software solution designed for demand forecasting. It incorporates AI techniques to enhance prediction accuracy and offers retailers insights into potential demand fluctuations.
Conclusion
As the retail landscape continues to evolve, leveraging AI for demand forecasting has become a necessity rather than a luxury. By implementing AI-driven tools and methodologies, retailers can gain a competitive edge, optimize their operations, and ultimately enhance customer satisfaction. Embracing these technologies not only streamlines processes but also empowers businesses to make data-driven decisions that foster growth and resilience in an ever-changing market.
Keyword: AI demand forecasting retail industry