AI Driven Document Analysis in Drug Development Optimization
Topic: AI Summarizer Tools
Industry: Pharmaceuticals
Discover how AI-driven document analysis enhances drug development efficiency accuracy and cost-effectiveness in the pharmaceutical industry
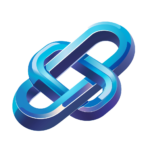
Optimizing Drug Development with AI-Driven Document Analysis
The Role of AI in Pharmaceuticals
Artificial Intelligence (AI) has emerged as a transformative force in the pharmaceutical industry, particularly in the realm of drug development. The complexity of drug research, coupled with the vast amount of documentation generated throughout the process, necessitates innovative solutions. AI-driven document analysis tools are streamlining workflows, enhancing data accuracy, and accelerating time-to-market for new therapies.
Understanding AI Summarizer Tools
AI summarizer tools leverage natural language processing (NLP) to analyze, interpret, and condense large volumes of text. In the context of pharmaceuticals, these tools can sift through clinical trial reports, research papers, regulatory documents, and other critical data sources to extract pertinent information quickly and efficiently.
Key Benefits of AI Summarizer Tools
- Enhanced Efficiency: By automating the summarization of lengthy documents, AI tools significantly reduce the time researchers spend on data extraction and analysis.
- Improved Accuracy: AI systems can minimize human error, ensuring that critical information is not overlooked during the document review process.
- Cost-Effective: Reducing the time and manpower required for document analysis translates into lower operational costs for pharmaceutical companies.
Implementing AI in Drug Development
To effectively implement AI-driven document analysis in drug development, organizations can adopt a multi-faceted approach that includes integrating specific AI tools into their existing workflows.
Examples of AI-Driven Products
Several AI-driven products are making waves in the pharmaceutical sector:
1. IBM Watson for Drug Discovery
IBM Watson utilizes AI to analyze scientific literature and clinical trial data, helping researchers identify potential drug candidates and biomarkers. By summarizing relevant findings, Watson enables faster decision-making and enhances the overall research process.
2. Elsevier’s PharmaPendium
This tool provides access to FDA-approved drug labels and summaries of clinical trials. Utilizing AI, PharmaPendium allows researchers to quickly navigate through extensive data, ensuring they have the critical information needed for informed decision-making.
3. BioSymetrics
BioSymetrics employs AI to streamline the drug discovery process by analyzing diverse datasets. Its document analysis capabilities help researchers focus on the most relevant studies and findings, thereby accelerating the identification of viable drug candidates.
Challenges and Considerations
While the benefits of AI-driven document analysis are substantial, organizations must also consider potential challenges. Data privacy, regulatory compliance, and the need for high-quality training data are critical factors that must be addressed to ensure successful implementation. Additionally, fostering a culture of innovation and providing adequate training for staff can facilitate a smoother transition to AI-enhanced workflows.
The Future of Drug Development
As the pharmaceutical industry continues to evolve, the integration of AI-driven document analysis tools will play a pivotal role in optimizing drug development processes. By harnessing the power of AI, companies can enhance their research capabilities, reduce time-to-market, and ultimately improve patient outcomes. The future of drug development is not just about discovering new medicines; it’s about doing so more efficiently and effectively through the strategic application of technology.
Keyword: AI document analysis in pharmaceuticals