AI Driven Hypothesis Generation Transforming Experimental Design
Topic: AI Language Tools
Industry: Research and Development
Discover how AI-driven hypothesis generation transforms experimental design in research enhancing efficiency and fostering innovation in scientific inquiry
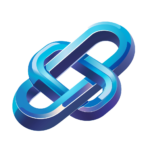
AI-Driven Hypothesis Generation: A Game-Changer for Experimental Design
Understanding the Role of AI in Research and Development
In the rapidly evolving landscape of research and development, artificial intelligence (AI) is emerging as a crucial player. The ability to analyze vast amounts of data quickly and accurately has led to significant advancements in various fields. One of the most compelling applications of AI in this domain is in hypothesis generation, which can fundamentally transform the way experiments are designed and executed.
The Importance of Hypothesis Generation
Hypothesis generation is a critical step in the scientific method, serving as the foundation for experimental design. A well-formulated hypothesis not only guides the research process but also enhances the likelihood of obtaining meaningful results. Traditionally, this process has relied heavily on human intuition and expertise, which can be time-consuming and prone to bias. However, AI-driven tools are now providing researchers with innovative ways to streamline and enhance this process.
How AI Can Be Implemented in Hypothesis Generation
The integration of AI into hypothesis generation can be achieved through various methodologies, including natural language processing (NLP), machine learning, and data mining. These technologies enable researchers to analyze existing literature, extract relevant information, and generate novel hypotheses based on identifiable patterns and trends.
Natural Language Processing (NLP)
NLP allows AI systems to interpret and generate human language, making it an invaluable tool for researchers. By utilizing NLP algorithms, researchers can sift through thousands of research papers, extracting pertinent data and identifying gaps in knowledge. This capability not only accelerates the hypothesis generation process but also ensures that new hypotheses are grounded in existing scientific understanding.
Machine Learning Techniques
Machine learning algorithms can analyze complex datasets to uncover correlations and causal relationships that may not be immediately apparent. By training models on historical data, these algorithms can predict potential outcomes and suggest hypotheses that researchers might not have considered. This predictive capability is particularly beneficial in fields such as drug discovery, where the identification of new therapeutic targets is crucial.
Examples of AI-Driven Tools for Research
Several AI-driven products and tools are currently available that facilitate hypothesis generation and enhance experimental design. Below are a few notable examples:
1. IBM Watson Discovery
IBM Watson Discovery leverages NLP to analyze unstructured data from various sources, including research papers, clinical trial data, and patents. By identifying trends and extracting insights, it enables researchers to formulate hypotheses based on comprehensive data analysis.
2. Elsevier’s SciVal
SciVal is a research analytics tool that uses machine learning to provide insights into research performance and trends. It allows researchers to visualize data and identify emerging areas of interest, facilitating hypothesis generation based on current research trajectories.
3. BioSymphony
BioSymphony focuses on the life sciences, utilizing AI to analyze biological data and generate hypotheses related to gene expression, protein interactions, and disease mechanisms. Its ability to integrate diverse datasets makes it a powerful tool for researchers aiming to uncover new biological insights.
Conclusion: The Future of Experimental Design
As AI technology continues to advance, its role in hypothesis generation will only become more significant. By harnessing the power of AI-driven tools, researchers can enhance their experimental design processes, reduce time spent on preliminary research, and ultimately drive innovation. The integration of AI into research not only streamlines hypothesis generation but also fosters a more data-driven approach to scientific inquiry, paving the way for groundbreaking discoveries.
Keyword: AI hypothesis generation tools