AI Driven Predictive Maintenance for Enhanced Manufacturing Uptime
Topic: AI Collaboration Tools
Industry: Manufacturing and Industrial Production
Discover how AI-driven predictive maintenance enhances manufacturing efficiency by predicting equipment failures and fostering collaboration for improved uptime
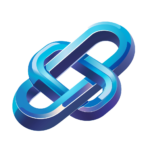
AI-Driven Predictive Maintenance: Collaborating for Better Uptime
The Importance of Predictive Maintenance in Manufacturing
In the fast-paced world of manufacturing and industrial production, maintaining operational efficiency is critical. Downtime can lead to significant financial losses, making predictive maintenance a key strategy for organizations aiming to enhance productivity. By leveraging artificial intelligence (AI), manufacturers can anticipate equipment failures before they occur, thereby minimizing disruptions and optimizing maintenance schedules.
Understanding AI-Driven Predictive Maintenance
Predictive maintenance employs data analysis tools and techniques to detect anomalies in equipment performance and predict potential failures. AI enhances this process by analyzing vast amounts of data collected from machinery, sensors, and operational logs. This predictive capability allows for timely interventions, reducing the likelihood of unexpected breakdowns.
How AI Can Be Implemented in Predictive Maintenance
Implementing AI-driven predictive maintenance involves several key steps:
- Data Collection: Gather data from various sources, including IoT sensors, machine logs, and historical maintenance records.
- Data Analysis: Utilize AI algorithms to analyze the collected data, identifying patterns and anomalies that may indicate potential failures.
- Model Development: Develop predictive models that can forecast equipment failures based on historical data and real-time inputs.
- Integration: Integrate predictive maintenance tools with existing manufacturing systems to enable seamless operation and real-time monitoring.
- Continuous Improvement: Continuously refine predictive models based on new data and feedback to enhance accuracy and reliability.
Examples of AI-Driven Tools for Predictive Maintenance
Several AI-driven tools and platforms are available to assist manufacturers in implementing predictive maintenance:
1. IBM Watson IoT
IBM Watson IoT offers a comprehensive suite of AI tools designed for predictive maintenance. By connecting devices and analyzing data, Watson can provide insights into equipment performance, enabling manufacturers to schedule maintenance proactively.
2. Siemens MindSphere
Siemens MindSphere is an industrial IoT platform that allows manufacturers to collect and analyze data from their production processes. With built-in AI capabilities, MindSphere helps organizations predict equipment failures and optimize maintenance schedules.
3. GE Digital’s Predix
GE Digital’s Predix platform is tailored for industrial applications, providing advanced analytics and machine learning capabilities. Predix enables manufacturers to monitor asset health in real time, predicting failures before they impact production.
4. Microsoft Azure IoT
Microsoft Azure IoT provides a robust framework for building predictive maintenance solutions. By leveraging machine learning algorithms, organizations can analyze data from connected devices and implement predictive maintenance strategies effectively.
Collaboration for Enhanced Uptime
AI-driven predictive maintenance is not just about technology; it also involves collaboration among various stakeholders. Effective communication between maintenance teams, operations staff, and IT professionals is essential for successful implementation. By fostering a culture of collaboration, organizations can ensure that insights derived from AI analytics are acted upon promptly, leading to improved uptime and operational efficiency.
Conclusion
As the manufacturing landscape continues to evolve, AI-driven predictive maintenance will play a pivotal role in enhancing operational performance. By leveraging advanced AI tools and fostering collaboration among teams, organizations can achieve better uptime, reduce costs, and maintain a competitive edge in the industry. Embracing these technologies is not merely an option; it is a necessity for those looking to thrive in an increasingly data-driven world.
Keyword: AI predictive maintenance solutions