AI Driven Predictive Maintenance for Smarter Factory Equipment
Topic: AI Design Tools
Industry: Manufacturing
Discover how AI-driven predictive maintenance enhances factory equipment efficiency reduces downtime and saves costs for manufacturers today.
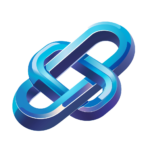
AI-Driven Predictive Maintenance: Designing Smarter Factory Equipment
Understanding Predictive Maintenance
Predictive maintenance is an advanced approach that leverages data analysis to predict equipment failures before they occur. By utilizing artificial intelligence (AI), manufacturers can enhance their operational efficiency, reduce downtime, and ultimately save costs. The integration of AI in predictive maintenance marks a significant shift from traditional maintenance strategies, which often rely on scheduled inspections and reactive measures.
The Role of AI in Predictive Maintenance
AI plays a pivotal role in transforming maintenance practices by enabling the collection and analysis of vast amounts of data generated by factory equipment. Machine learning algorithms can identify patterns and anomalies that may indicate potential failures. This proactive approach allows manufacturers to schedule maintenance activities at optimal times, minimizing disruptions and maximizing equipment lifespan.
Key Components of AI-Driven Predictive Maintenance
- Data Acquisition: Sensors and IoT devices collect real-time data from machinery, including temperature, vibration, and operational speed.
- Data Processing: AI algorithms process the collected data to identify trends and predict potential failures.
- Decision Making: Insights derived from data analysis inform maintenance schedules and operational adjustments.
Implementing AI in Manufacturing
Integrating AI-driven predictive maintenance into manufacturing processes requires a strategic approach. Companies must ensure they have the right infrastructure in place, including robust data collection systems and analytics tools. Here are some steps to effectively implement AI in predictive maintenance:
1. Assess Current Capabilities
Evaluate existing equipment and data collection methods to identify gaps and opportunities for improvement.
2. Invest in AI Tools
Invest in AI-driven tools that can analyze data and provide actionable insights. Several products on the market can facilitate this process:
- IBM Maximo: This asset management platform utilizes AI to enhance predictive maintenance capabilities, offering real-time insights and analytics.
- Uptake: A predictive analytics platform that helps manufacturers monitor equipment health and predict failures using machine learning algorithms.
- Siemens MindSphere: An open cloud-based IoT operating system that connects industrial machines and enables advanced analytics for predictive maintenance.
3. Train Your Workforce
It is crucial to equip your workforce with the necessary skills to utilize AI tools effectively. Training programs should focus on data interpretation, machine learning basics, and the specific tools being implemented.
Case Studies: Successful Implementation of AI-Driven Predictive Maintenance
General Electric (GE)
GE has successfully integrated AI-driven predictive maintenance into its manufacturing processes. By using advanced analytics and machine learning, GE has improved its equipment uptime and reduced maintenance costs significantly. Their digital wind farm initiative is a prime example, where predictive maintenance has enhanced turbine performance and operational efficiency.
Siemens
Siemens has also made strides in predictive maintenance through its use of MindSphere. By connecting its manufacturing equipment to the cloud, Siemens can analyze data in real-time, allowing for timely maintenance interventions and improved asset reliability.
Conclusion
The adoption of AI-driven predictive maintenance is no longer a futuristic concept; it is a present-day necessity for manufacturers aiming to stay competitive. By leveraging AI tools and technologies, companies can design smarter factory equipment that not only enhances operational efficiency but also drives significant cost savings. As the manufacturing landscape continues to evolve, embracing AI in predictive maintenance will be key to achieving sustainable growth and innovation.
Keyword: AI predictive maintenance solutions