AI Driven Predictive Maintenance for Telecom Network Uptime
Topic: AI Media Tools
Industry: Telecommunications
Discover how AI-driven predictive maintenance can reduce downtime in telecom networks enhance service reliability and improve customer satisfaction
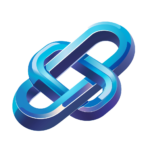
AI-Driven Predictive Maintenance: Reducing Downtime in Telecom Networks
Understanding Predictive Maintenance in Telecommunications
Predictive maintenance is a proactive approach to maintenance that leverages data analysis and artificial intelligence (AI) to predict equipment failures before they occur. In the telecommunications sector, where uptime is critical, implementing predictive maintenance strategies can significantly reduce downtime and enhance service reliability.
The Role of AI in Predictive Maintenance
Artificial intelligence plays a pivotal role in predictive maintenance by processing vast amounts of data from network equipment and identifying patterns that may indicate potential failures. By utilizing machine learning algorithms, telecom operators can analyze historical performance data, environmental factors, and usage patterns to forecast equipment malfunctions.
Key Benefits of AI-Driven Predictive Maintenance
- Reduced Downtime: By predicting failures ahead of time, telecom companies can schedule maintenance during off-peak hours, minimizing disruptions.
- Cost Savings: Preventive maintenance reduces the costs associated with emergency repairs and equipment replacements.
- Enhanced Customer Satisfaction: Maintaining network reliability leads to improved service quality, resulting in higher customer retention rates.
Implementing AI-Driven Predictive Maintenance
To effectively implement AI-driven predictive maintenance in telecom networks, companies can follow a structured approach:
1. Data Collection
The first step involves gathering data from various sources, including network devices, sensors, and customer interactions. This data serves as the foundation for predictive analytics.
2. Data Analysis
Once data is collected, AI algorithms can analyze it to identify trends and anomalies. Machine learning models, such as regression analysis and neural networks, can be employed to predict future equipment performance based on historical data.
3. Actionable Insights
The insights generated from data analysis can inform maintenance schedules and resource allocation, allowing telecom operators to address potential issues before they escalate.
4. Continuous Improvement
Implementing a feedback loop is crucial for refining predictive maintenance strategies. Continuous monitoring and updating of AI models ensure that predictions remain accurate and relevant.
Examples of AI-Driven Tools and Products
Several AI-driven tools and products are available to facilitate predictive maintenance in telecommunications:
1. IBM Watson IoT
IBM Watson IoT offers AI-powered analytics that can help telecom companies monitor network performance in real-time. By integrating with existing infrastructure, it provides actionable insights that enhance predictive maintenance efforts.
2. Siemens MindSphere
Siemens MindSphere is an open IoT operating system that connects machines and physical infrastructure to the digital world. It enables telecom operators to analyze data from equipment and optimize maintenance schedules based on predictive insights.
3. GE Digital’s Predix
GE Digital’s Predix platform is designed for industrial applications, including telecommunications. It utilizes machine learning to predict equipment failures and optimize maintenance processes, ultimately reducing unplanned downtime.
Conclusion
AI-driven predictive maintenance represents a transformative approach for the telecommunications industry. By leveraging advanced analytics and machine learning tools, telecom operators can significantly reduce downtime, enhance operational efficiency, and improve customer satisfaction. As the industry continues to evolve, embracing these technologies will be crucial for maintaining a competitive edge in a rapidly changing landscape.
Keyword: AI predictive maintenance telecom networks