AI Driven Supply Chain Management Reducing Food Waste Effectively
Topic: AI App Tools
Industry: Agriculture
Discover how AI-driven supply chain management can reduce food waste from farm to table by optimizing demand forecasting inventory and logistics efficiency
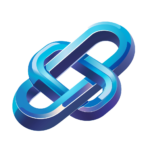
AI-Driven Supply Chain Management: Reducing Food Waste from Farm to Table
Understanding the Challenge of Food Waste
Food waste is a pressing global issue, with approximately one-third of all food produced for human consumption lost or wasted each year. This not only has significant economic implications but also contributes to environmental degradation. The supply chain, encompassing everything from agricultural production to distribution and consumption, plays a critical role in this challenge. However, with the advent of artificial intelligence (AI), there is a transformative opportunity to enhance supply chain management and significantly reduce food waste.
The Role of AI in Supply Chain Management
AI can optimize various aspects of the supply chain by enhancing decision-making processes, improving operational efficiency, and providing predictive analytics. By leveraging AI technologies, stakeholders in the agricultural sector can gain insights into demand forecasting, inventory management, and logistics optimization, all of which are crucial in minimizing food waste.
1. Predictive Analytics for Demand Forecasting
One of the primary ways AI can assist in supply chain management is through predictive analytics. By analyzing historical data and current market trends, AI algorithms can forecast demand more accurately. This helps farmers and distributors align production with actual market needs, reducing the likelihood of overproduction and subsequent waste.
For example, tools like IBM Watson utilize machine learning to analyze data from various sources, enabling more accurate demand predictions. This allows agricultural producers to adjust their planting schedules and quantities accordingly.
2. Inventory Optimization
AI-driven inventory management systems can significantly reduce waste by ensuring that perishable goods are distributed efficiently. By monitoring stock levels in real-time and predicting when items are likely to expire, these systems can automate reordering processes and alert stakeholders to items that need to be sold quickly.
Tools such as Blue Yonder use AI to optimize inventory across the supply chain, helping businesses maintain optimal stock levels while minimizing waste. This not only improves profitability but also supports sustainability efforts.
3. Smart Logistics and Transportation
Efficient logistics is essential for minimizing food waste, particularly in the transportation of perishable goods. AI can enhance route optimization, ensuring that products are delivered in the shortest time possible while maintaining quality. By analyzing traffic patterns, weather conditions, and delivery schedules, AI algorithms can suggest the most efficient routes.
For instance, Project44 offers an AI-driven platform that provides real-time visibility and predictive analytics for logistics operations. This enables businesses to respond swiftly to disruptions and optimize their transportation strategies, ultimately reducing the likelihood of spoilage during transit.
4. Quality Monitoring with AI
AI technologies can also be employed to monitor the quality of food products throughout the supply chain. Using computer vision and machine learning, AI systems can identify defects or spoilage in real-time, allowing for immediate intervention. This proactive approach helps ensure that only high-quality products reach consumers.
Companies like AgroStar are developing AI solutions that utilize image recognition to assess crop health and quality, enabling farmers to make informed decisions about harvesting and distribution.
Implementation Strategies for AI Tools
To effectively implement AI-driven tools for supply chain management, agricultural businesses should consider the following strategies:
1. Data Integration
Successful AI implementation relies on the availability of high-quality data. Businesses should invest in systems that integrate data from various sources, including weather forecasts, market trends, and historical sales data.
2. Collaboration Across the Supply Chain
Collaboration among all stakeholders, from farmers to retailers, is essential for maximizing the benefits of AI. Sharing data and insights can lead to more informed decision-making and improved supply chain efficiency.
3. Continuous Learning and Adaptation
AI systems thrive on continuous learning. Organizations should regularly update their AI models with new data to enhance accuracy and effectiveness. This adaptability is crucial in a rapidly changing agricultural landscape.
Conclusion
AI-driven supply chain management presents a significant opportunity to reduce food waste from farm to table. By implementing predictive analytics, optimizing inventory, enhancing logistics, and monitoring quality, agricultural stakeholders can make informed decisions that contribute to sustainability and profitability. As the agricultural sector continues to embrace AI technologies, the potential for reducing food waste and improving overall efficiency will only grow.
Keyword: AI supply chain food waste reduction