AI Enabled Edge Computing Transforming IoT Network Architecture
Topic: AI Networking Tools
Industry: Information Technology
Discover how AI-enabled edge computing is revolutionizing IoT network architecture with reduced latency enhanced security and real-time data analysis
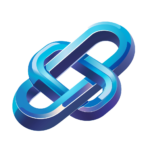
AI-Enabled Edge Computing: Reshaping Network Architecture for IoT
The Intersection of AI and Edge Computing
As the Internet of Things (IoT) continues to proliferate, the demand for efficient network architecture has never been more critical. Edge computing, which processes data closer to the source rather than relying solely on centralized data centers, is emerging as a vital component of this infrastructure. The integration of artificial intelligence (AI) into edge computing is reshaping how organizations manage and utilize their networks, providing enhanced capabilities and efficiencies.
Understanding AI-Enabled Edge Computing
AI-enabled edge computing combines the processing power of AI with the distributed nature of edge computing. This synergy allows for real-time data analysis, predictive maintenance, and intelligent decision-making at the edge of the network. By leveraging AI, organizations can optimize their IoT deployments, reduce latency, and improve overall performance.
Key Benefits of AI-Enabled Edge Computing
- Reduced Latency: By processing data closer to the source, AI-enabled edge computing minimizes the time it takes to analyze and respond to data inputs.
- Bandwidth Efficiency: With AI algorithms filtering and processing data at the edge, only relevant information is sent to the cloud, conserving bandwidth.
- Enhanced Security: AI can identify anomalies and potential threats in real-time, allowing for proactive security measures at the edge.
- Scalability: Organizations can easily scale their IoT solutions as AI tools can adapt to varying data loads and processing requirements.
Implementing AI in Edge Computing
The implementation of AI in edge computing requires a thoughtful approach, including the selection of appropriate tools and platforms that align with organizational goals.
AI-Driven Tools and Products
Several innovative tools and products are available to facilitate the integration of AI into edge computing environments:
1. NVIDIA Jetson
NVIDIA Jetson is a powerful AI computing platform designed for edge devices. It enables developers to deploy deep learning and computer vision applications in real-time, making it ideal for smart cameras and autonomous machines.
2. Microsoft Azure IoT Edge
Azure IoT Edge extends cloud intelligence to edge devices, allowing organizations to run AI algorithms locally. This platform supports various AI frameworks, enabling seamless integration with existing IoT solutions.
3. Google Coral
Google Coral provides a set of tools and hardware designed for building AI applications at the edge. With its Edge TPU, developers can create low-latency, high-performance machine learning models for IoT devices.
4. AWS IoT Greengrass
AWS IoT Greengrass allows users to run local compute, messaging, and data caching for connected devices. By integrating AI capabilities, organizations can process data closer to the source and make real-time decisions based on AI predictions.
Real-World Applications of AI-Enabled Edge Computing
Numerous industries are already harnessing the power of AI-enabled edge computing to drive innovation and efficiency:
Manufacturing
In manufacturing, AI-driven edge devices can monitor machinery performance, predict failures, and optimize maintenance schedules, significantly reducing downtime and operational costs.
Healthcare
Healthcare providers are utilizing AI-enabled edge computing to analyze patient data in real-time, improving diagnostics and enabling remote monitoring of patients through wearable devices.
Smart Cities
Smart city initiatives leverage AI at the edge to manage traffic flow, monitor environmental conditions, and enhance public safety through intelligent surveillance systems.
The Future of AI-Enabled Edge Computing
The convergence of AI and edge computing is set to transform network architecture for IoT, allowing organizations to unlock new levels of efficiency, scalability, and security. As AI technologies continue to advance, the potential for innovative applications in various sectors will expand, driving further investment and interest in AI-enabled edge computing solutions.
Conclusion
AI-enabled edge computing is not merely a trend; it represents a fundamental shift in how organizations approach network architecture for IoT. By adopting AI-driven tools and embracing the capabilities of edge computing, businesses can position themselves at the forefront of technological advancement, ready to meet the challenges of an increasingly connected world.
Keyword: AI edge computing solutions