AI Enhances Data Quality and Integrity in Clinical Trials
Topic: AI Health Tools
Industry: Clinical trial management companies
Discover how AI enhances data quality and integrity in clinical trials through automation predictive analytics and real-time monitoring for reliable results
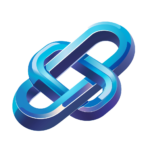
The Role of AI in Enhancing Data Quality and Integrity in Clinical Trials
Introduction to AI in Clinical Trials
In the rapidly evolving landscape of clinical trials, the integration of artificial intelligence (AI) is becoming increasingly vital. Clinical trial management companies are leveraging AI health tools to enhance data quality and integrity, ensuring that the results are not only reliable but also actionable. This article explores how AI can be effectively implemented in clinical trials, highlighting specific tools and AI-driven products that are paving the way for improved data management.
Understanding Data Quality and Integrity
Data quality refers to the accuracy, completeness, and reliability of data collected during clinical trials. Integrity, on the other hand, pertains to the consistency and trustworthiness of this data over time. Both elements are crucial for regulatory compliance and for the overall success of clinical trials. AI technologies can significantly bolster these aspects by automating processes, reducing human error, and providing advanced analytics capabilities.
AI Implementation in Clinical Trials
Implementing AI in clinical trials involves several key strategies:
1. Data Cleaning and Validation
AI algorithms can automatically identify and rectify inconsistencies or anomalies in data sets. Tools such as IBM Watson Health utilize machine learning to enhance data cleaning processes, ensuring that only high-quality data is used for analysis. By automating these tasks, clinical trial management companies can save time and resources while improving data accuracy.
2. Predictive Analytics
Predictive analytics powered by AI can forecast potential outcomes and identify trends in clinical trial data. For instance, Medidata Solutions offers AI-driven analytics that help researchers predict patient enrollment rates and drop-out risks, allowing for better planning and resource allocation. This proactive approach enhances data integrity by ensuring that trials are conducted under optimal conditions.
3. Real-Time Monitoring
AI tools enable real-time monitoring of trial data, which is essential for maintaining data integrity. Platforms like Oracle’s Siebel Clinical incorporate AI to provide continuous oversight of trial data, flagging any discrepancies as they occur. This immediate feedback loop allows for timely interventions, thus safeguarding the quality of the data collected.
4. Enhanced Patient Engagement
AI can also improve patient engagement, which is crucial for data integrity. Tools such as TrialX use AI to personalize communication with participants, ensuring that they remain informed and motivated throughout the trial process. Enhanced engagement leads to better adherence to protocols, ultimately improving the quality of the data collected.
Examples of AI-Driven Products in Clinical Trials
Several AI-driven products are making significant strides in clinical trial management:
1. Deep 6 AI
Deep 6 AI utilizes natural language processing to analyze unstructured data from various sources, including clinical notes and electronic health records. This capability allows researchers to identify suitable candidates for trials more efficiently, thereby enhancing the quality of the participant pool.
2. CluePoints
CluePoints employs AI to provide risk-based monitoring solutions that assess data quality and integrity across clinical trials. By identifying potential risks early in the trial process, CluePoints helps organizations address issues proactively, ensuring that data remains reliable throughout the study.
3. Antidote
Antidote leverages AI to match patients with clinical trials based on their specific health conditions and preferences. This targeted approach not only improves recruitment efficiency but also enhances the quality of data collected by ensuring that participants are well-suited for the study.
Conclusion
The integration of AI in clinical trials represents a transformative shift towards enhancing data quality and integrity. By adopting AI-driven tools and technologies, clinical trial management companies can streamline operations, reduce errors, and ultimately deliver more reliable results. As the industry continues to evolve, the role of AI will undoubtedly become more central, driving innovation and improving patient outcomes in clinical research.
Keyword: AI in clinical trials data quality