AI Image Tools vs Traditional Methods Cost Benefits for Manufacturers
Topic: AI Image Tools
Industry: Manufacturing
Discover the cost benefits of AI image tools vs traditional methods in manufacturing Enhance accuracy speed and efficiency while reducing labor costs
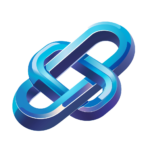
AI Image Tools vs. Traditional Methods: Cost-Benefit Analysis for Manufacturers
Introduction to AI Image Tools in Manufacturing
In recent years, the manufacturing sector has witnessed a significant transformation driven by advancements in artificial intelligence (AI). Among these innovations, AI image tools have emerged as a game-changer, offering manufacturers enhanced capabilities for quality control, defect detection, and process optimization. This article delves into a cost-benefit analysis of AI image tools compared to traditional methods, providing insights into their implementation and specific examples of tools available in the market.
Understanding Traditional Methods
Traditionally, manufacturers have relied on manual inspections and conventional imaging techniques for quality assurance. These methods often involve human inspectors visually examining products or using basic imaging systems to identify defects. While effective to some extent, traditional methods can be labor-intensive, time-consuming, and prone to human error.
Limitations of Traditional Methods
- Labor Costs: Manual inspections require a significant workforce, increasing operational costs.
- Inconsistency: Human inspectors may have varying levels of expertise, leading to inconsistent quality assessments.
- Time-Consuming: Traditional methods often slow down production lines, impacting overall efficiency.
Advantages of AI Image Tools
AI image tools leverage advanced algorithms and machine learning to automate the inspection process, offering a range of benefits that can significantly enhance manufacturing operations.
Cost Efficiency
One of the primary advantages of implementing AI image tools is the reduction in labor costs. By automating inspections, manufacturers can minimize the need for a large workforce, reallocating resources to more value-added activities.
Increased Accuracy
AI-driven systems utilize deep learning techniques to analyze images with a high degree of precision. For instance, tools like Google Cloud Vision and Amazon Rekognition can accurately identify defects or anomalies that may be missed by the human eye, ensuring consistent quality across production lines.
Speed and Efficiency
AI image tools can process images at a much faster rate than manual inspections. This increased speed translates to quicker turnaround times and enhanced productivity, allowing manufacturers to meet market demands more effectively.
Implementing AI Image Tools in Manufacturing
The implementation of AI image tools involves several key steps to ensure a smooth transition from traditional methods.
1. Assess Current Processes
Manufacturers should begin by evaluating their existing quality control processes to identify areas where AI image tools can add value.
2. Select the Right Tools
Choosing the appropriate AI image tools is crucial. Options such as Siemens’ MindSphere and Cognex VisionPro provide robust platforms for integrating AI capabilities into manufacturing workflows.
3. Train Staff
Training staff on how to use these new tools is essential for maximizing their potential. Manufacturers should invest in training programs to ensure employees are well-versed in operating AI systems.
4. Monitor and Optimize
Once implemented, it is vital to continuously monitor the performance of AI image tools and optimize processes based on real-time data analytics.
Case Studies of Successful Implementation
Several manufacturers have successfully adopted AI image tools, reaping substantial benefits.
Example 1: BMW
BMW has integrated AI-driven image recognition systems in its production lines to enhance quality control. By utilizing tools from Cognex, BMW has reduced inspection times by over 30%, significantly improving overall efficiency.
Example 2: Procter & Gamble
Procter & Gamble employs AI image analysis to monitor product quality in real-time. By leveraging Microsoft Azure’s AI capabilities, the company has achieved a notable decrease in defect rates, ultimately saving costs associated with product recalls.
Conclusion
The transition from traditional methods to AI image tools in manufacturing presents a compelling opportunity for cost savings, improved accuracy, and enhanced efficiency. As manufacturers continue to navigate the complexities of modern production environments, embracing AI technology will be crucial in maintaining a competitive edge. By carefully assessing their needs and implementing the right tools, manufacturers can harness the power of AI to drive innovation and success.
Keyword: AI image tools for manufacturing