AI in Clinical Decision Support Enhancing Doctor Decision Making
Topic: AI App Tools
Industry: Healthcare
Discover how AI enhances clinical decision support systems aiding doctors in real-time for better patient outcomes and efficient healthcare delivery
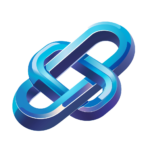
AI in Clinical Decision Support: Apps Assisting Doctors in Real-Time
Understanding Clinical Decision Support Systems (CDSS)
Clinical Decision Support Systems (CDSS) are integrated tools designed to enhance healthcare delivery by providing clinicians with knowledge and patient-specific information, intelligently filtered and presented at appropriate times. The integration of artificial intelligence (AI) into these systems has revolutionized the way healthcare professionals make decisions, ultimately improving patient outcomes and operational efficiency.
The Role of AI in Healthcare
Artificial intelligence has the potential to transform healthcare by analyzing vast amounts of data, identifying patterns, and generating insights that would be difficult for human clinicians to discern. AI can assist in various areas, including diagnostics, treatment recommendations, and predictive analytics, allowing for more informed and timely clinical decisions.
Implementing AI in Clinical Decision Support
To implement AI effectively in clinical decision support, healthcare organizations must focus on several key areas:
- Data Integration: Seamless integration of electronic health records (EHRs) and other data sources is crucial for AI algorithms to access relevant patient information.
- Algorithm Development: Collaborating with data scientists and clinicians to develop algorithms that can analyze patient data and provide actionable insights is essential.
- User-Friendly Interfaces: Ensuring that AI tools are easy to use and integrate into existing workflows will encourage adoption among healthcare professionals.
- Continuous Learning: AI systems should be designed to learn from new data and outcomes, continually improving their recommendations over time.
Examples of AI-Driven Tools in Clinical Decision Support
Several AI-driven applications are currently making significant strides in clinical decision support, enhancing the capabilities of healthcare professionals in real-time:
1. IBM Watson for Oncology
IBM Watson for Oncology utilizes natural language processing and machine learning to analyze medical literature, clinical trial data, and patient records. By providing evidence-based treatment recommendations, it aids oncologists in making informed decisions tailored to individual patient needs.
2. Aidoc
Aidoc is an AI-powered radiology tool that assists radiologists in identifying critical conditions in medical images. By flagging abnormalities in real-time, Aidoc enables faster diagnosis and treatment, ultimately improving patient outcomes in emergency settings.
3. Zebra Medical Vision
Zebra Medical Vision offers a suite of AI algorithms that analyze medical imaging data to detect various conditions, such as cardiovascular diseases and cancers. By providing radiologists with automated insights, the tool helps prioritize cases that require immediate attention.
4. ClinicalKey
ClinicalKey is a clinical search engine that leverages AI to provide healthcare professionals with the most relevant information and clinical guidelines. Its ability to deliver evidence-based content in real-time supports clinicians in making informed decisions during patient consultations.
5. Tempus
Tempus utilizes AI to analyze clinical and molecular data, enabling personalized treatment plans for cancer patients. By integrating genomic data with clinical outcomes, Tempus helps oncologists make data-driven decisions that enhance patient care.
Challenges and Considerations
While the integration of AI in clinical decision support offers numerous benefits, several challenges must be addressed:
- Data Privacy: Ensuring patient data privacy and compliance with regulations such as HIPAA is paramount when utilizing AI technologies.
- Bias in Algorithms: AI systems can inadvertently perpetuate biases present in training data, leading to unequal treatment recommendations. Continuous monitoring and adjustment are necessary to mitigate this risk.
- Training and Adoption: Clinicians must be trained to use AI tools effectively, and organizations must foster a culture of acceptance to encourage their integration into everyday practice.
Conclusion
The implementation of AI in clinical decision support systems represents a significant advancement in healthcare technology. By leveraging AI-driven tools, healthcare professionals can enhance their decision-making capabilities, ultimately leading to improved patient outcomes. As the technology continues to evolve, it is essential for healthcare organizations to address the associated challenges, ensuring that AI serves as a valuable ally in the pursuit of better healthcare delivery.
Keyword: AI clinical decision support tools