AI in Clinical Decision Support Enhancing Evidence Based Practice
Topic: AI Health Tools
Industry: Medical research institutions
Discover how AI enhances clinical decision support in healthcare by improving evidence-based practice and patient outcomes through advanced algorithms and data integration
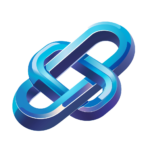
AI in Clinical Decision Support: Enhancing Evidence-Based Practice
Introduction to AI in Clinical Decision Support
Artificial Intelligence (AI) is transforming various sectors, and healthcare is no exception. In medical research institutions, AI is increasingly being integrated into clinical decision support (CDS) systems, enhancing evidence-based practice and improving patient outcomes. By leveraging large datasets and advanced algorithms, AI tools can assist healthcare professionals in making informed decisions based on the latest research and clinical guidelines.
Implementation of AI in Clinical Decision Support
The successful implementation of AI in clinical decision support requires a strategic approach that encompasses data collection, algorithm development, integration with existing systems, and continuous evaluation. Here are key steps to consider:
1. Data Collection and Management
AI algorithms rely on high-quality data to produce accurate insights. Medical research institutions must prioritize the collection and management of comprehensive datasets, including patient demographics, clinical histories, and treatment outcomes. Ensuring data integrity and compliance with regulations such as HIPAA is crucial.
2. Algorithm Development
Developing robust AI algorithms involves collaboration between data scientists, clinicians, and researchers. Machine learning techniques can be employed to analyze historical data and identify patterns that inform clinical decision-making. For instance, predictive models can forecast patient deterioration or treatment responses based on previous cases.
3. Integration with Clinical Workflows
AI tools must seamlessly integrate with existing electronic health record (EHR) systems to be effective. This allows healthcare professionals to access AI-generated insights without disrupting their workflow. User-friendly interfaces and real-time data processing are essential for maximizing adoption among clinicians.
4. Continuous Evaluation and Improvement
Regular evaluation of AI systems is necessary to ensure their effectiveness and accuracy. Feedback from healthcare providers can guide iterative improvements, helping to refine algorithms and enhance their applicability in clinical settings.
Examples of AI-Driven Tools in Clinical Decision Support
Several AI-driven products are currently making significant strides in clinical decision support. Below are notable examples that exemplify the potential of AI in enhancing evidence-based practice:
1. IBM Watson for Oncology
IBM Watson for Oncology utilizes natural language processing and machine learning to analyze vast amounts of medical literature and patient data. It provides oncologists with evidence-based treatment recommendations tailored to individual patient profiles, thereby improving the quality of cancer care.
2. Aidoc
Aidoc is an AI-powered radiology platform that assists radiologists in detecting abnormalities in medical imaging. By prioritizing cases that require immediate attention, Aidoc enhances the efficiency of radiological workflows and supports timely clinical decisions.
3. Zebra Medical Vision
Zebra Medical Vision offers AI algorithms that analyze medical imaging data to identify a wide range of conditions, from cardiovascular diseases to cancers. By providing radiologists with automated insights, the platform aids in making faster and more accurate diagnoses.
4. Tempus
Tempus leverages AI and machine learning to analyze clinical and molecular data, enabling personalized treatment plans for cancer patients. By integrating genomic information with clinical data, Tempus enhances the precision of treatment recommendations and supports oncologists in their decision-making processes.
Challenges and Considerations
While the benefits of integrating AI into clinical decision support are evident, several challenges must be addressed:
1. Data Privacy and Security
Ensuring the privacy and security of patient data is paramount. Medical research institutions must implement robust security measures to protect sensitive information from breaches and unauthorized access.
2. Ethical Considerations
The use of AI in healthcare raises ethical questions regarding accountability, bias, and transparency. Institutions must establish ethical guidelines to govern AI applications, ensuring that they are used responsibly and equitably.
3. Training and Adoption
For AI tools to be effective, healthcare professionals must receive adequate training on their use. Institutions should invest in educational programs to familiarize clinicians with AI technologies and their potential benefits.
Conclusion
AI in clinical decision support represents a significant advancement in enhancing evidence-based practice within medical research institutions. By implementing AI-driven tools, healthcare professionals can make more informed decisions, ultimately leading to improved patient outcomes. As the technology continues to evolve, it is essential for institutions to address the associated challenges and ensure that AI is utilized ethically and effectively in clinical settings.
Keyword: AI clinical decision support tools