AI in Pharma Manufacturing Optimizing Supply Chains and Quality Control
Topic: AI Search Tools
Industry: Pharmaceuticals and Biotechnology
Discover how AI is revolutionizing pharma manufacturing by optimizing supply chains and enhancing quality control for greater efficiency and cost savings
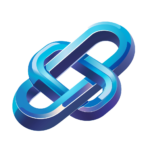
The Rise of AI in Pharma Manufacturing: Optimizing Supply Chains and Quality Control
Introduction to AI in Pharmaceuticals
Artificial Intelligence (AI) is transforming various industries, and the pharmaceutical sector is no exception. As companies strive to enhance efficiency and reduce costs, AI technologies are increasingly being integrated into manufacturing processes. This article explores how AI is optimizing supply chains and quality control in pharma manufacturing, highlighting specific tools and applications that are driving this change.
Enhancing Supply Chain Management
In the pharmaceutical industry, supply chain efficiency is critical. AI can significantly improve forecasting, inventory management, and logistics, ensuring that the right products are available at the right time.
Predictive Analytics
AI-driven predictive analytics tools, such as IBM Watson Supply Chain, leverage historical data to predict future demand trends. By analyzing patterns in sales data, these tools help manufacturers anticipate fluctuations in demand, allowing for better inventory management and reduced waste.
Smart Logistics
AI can also enhance logistics through tools like FourKites, which provides real-time visibility into supply chain operations. By utilizing machine learning algorithms, FourKites optimizes shipping routes and delivery schedules, reducing delays and improving overall efficiency.
Quality Control through AI
Quality control is paramount in pharmaceutical manufacturing, where even minor deviations can lead to significant consequences. AI technologies are being employed to ensure that products meet stringent quality standards.
Automated Quality Inspection
AI-powered inspection systems, such as Deep Learning-based Image Recognition, utilize computer vision to detect defects in products during the manufacturing process. These systems can analyze images of products at high speed, identifying anomalies that human inspectors might miss, thus ensuring higher quality standards.
Data-Driven Quality Assurance
AI tools like Qventus leverage machine learning to analyze data from various stages of production. By identifying trends and correlations in quality metrics, Qventus helps manufacturers implement proactive measures to mitigate potential quality issues before they arise.
Case Studies: Successful AI Implementation
Several pharmaceutical companies have successfully integrated AI into their manufacturing processes, yielding impressive results.
Case Study: Roche
Roche has implemented AI-driven analytics to optimize its supply chain operations. By utilizing predictive models, Roche has improved its inventory turnover rates and reduced stockouts, resulting in increased operational efficiency and cost savings.
Case Study: Novartis
Novartis has embraced AI for quality control by deploying machine learning algorithms to analyze production data. This initiative has led to a significant reduction in product defects, enhancing overall product quality and compliance with regulatory standards.
Challenges and Considerations
While the benefits of AI in pharma manufacturing are clear, several challenges must be addressed. Data privacy concerns, the need for robust cybersecurity measures, and the integration of AI systems with existing infrastructure are critical considerations for companies looking to adopt AI technologies.
Conclusion
The rise of AI in pharmaceutical manufacturing presents a significant opportunity for companies to optimize their supply chains and enhance quality control. By leveraging AI-driven tools and technologies, manufacturers can achieve greater efficiency, reduce costs, and ultimately provide better products to patients. As the industry continues to evolve, embracing AI will be essential for staying competitive in a rapidly changing landscape.
Keyword: AI in pharmaceutical manufacturing