AI in Post-Market Drug Surveillance for Enhanced Safety
Topic: AI Data Tools
Industry: Pharmaceuticals
Discover how AI enhances post-market drug surveillance by improving safety monitoring and real-world evidence analysis for better healthcare outcomes.
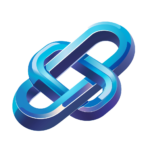
AI and Real-World Evidence: Enhancing Post-Market Drug Surveillance
Understanding the Importance of Post-Market Drug Surveillance
Post-market drug surveillance is a critical component of pharmaceutical safety and efficacy. As new drugs enter the market, it is essential to monitor their performance in real-world settings to identify any potential adverse effects or unforeseen interactions. This ongoing assessment helps ensure that medications remain safe for public use and informs regulatory decisions regarding drug approvals and market withdrawals.
The Role of Artificial Intelligence in Post-Market Surveillance
Artificial intelligence (AI) is revolutionizing the way pharmaceutical companies approach post-market drug surveillance. By leveraging advanced data analytics and machine learning algorithms, AI can enhance the collection, analysis, and interpretation of real-world evidence (RWE). This allows for more efficient monitoring of drug performance and patient outcomes, ultimately leading to improved safety profiles and better-informed healthcare decisions.
Key AI Applications in Post-Market Drug Surveillance
Several AI-driven tools and methodologies can be employed to enhance post-market drug surveillance:
1. Natural Language Processing (NLP)
NLP technologies can process vast amounts of unstructured data from sources such as electronic health records, social media, and patient forums. By analyzing this data, NLP can identify patterns and trends related to drug safety and efficacy. For instance, tools like IBM Watson can sift through millions of clinical notes and reports to detect adverse events associated with specific medications.
2. Predictive Analytics
Predictive analytics utilizes machine learning algorithms to forecast potential adverse events based on historical data. By analyzing patient demographics, comorbidities, and treatment regimens, AI can identify high-risk populations and predict which patients may experience negative outcomes. Platforms such as SAS Viya offer predictive modeling capabilities that can be tailored for drug safety assessments.
3. Automated Signal Detection
AI can automate the signal detection process, which involves identifying unusual patterns that may indicate safety concerns. Tools like Oracle’s Argus Safety employ AI algorithms to monitor and analyze adverse event reports in real-time, allowing for rapid identification of potential safety signals. This proactive approach can significantly reduce the time it takes to respond to emerging safety issues.
4. Data Integration and Visualization
AI-driven data integration platforms can aggregate data from multiple sources, including clinical trials, electronic health records, and patient registries. By providing a comprehensive view of drug performance, these platforms facilitate better decision-making. Tools like Tableau and Power BI can visualize this integrated data, making it easier for stakeholders to interpret findings and take action when necessary.
Case Studies: Successful Implementation of AI in Drug Surveillance
Several pharmaceutical companies have successfully implemented AI tools to enhance their post-market surveillance efforts:
Case Study 1: Pfizer
Pfizer has utilized AI-driven analytics to monitor the safety of its vaccines. By employing machine learning algorithms to analyze data from diverse sources, the company has been able to identify potential safety signals more quickly than traditional methods allow.
Case Study 2: Johnson & Johnson
Johnson & Johnson has integrated NLP tools into its pharmacovigilance processes. By analyzing social media conversations and patient-reported outcomes, the company has gained valuable insights into real-world drug performance, enabling timely interventions when safety concerns arise.
Challenges and Considerations
While the integration of AI in post-market drug surveillance presents numerous advantages, it is not without challenges. Data privacy concerns, regulatory compliance, and the need for high-quality data remain significant hurdles. Pharmaceutical companies must navigate these issues carefully to ensure the ethical and effective use of AI technologies.
Conclusion
Artificial intelligence holds immense potential to enhance post-market drug surveillance through improved data analysis, predictive capabilities, and real-time monitoring. By adopting AI-driven tools and methodologies, pharmaceutical companies can ensure greater drug safety, ultimately benefiting patients and healthcare systems alike. As the industry continues to evolve, the integration of AI into drug surveillance will likely become a standard practice, paving the way for more efficient and effective healthcare solutions.
Keyword: AI in post-market drug surveillance