AI in Predictive Maintenance for Food Manufacturing Reduces Downtime
Topic: AI Food Tools
Industry: Food Manufacturing
Discover how AI-driven predictive maintenance in food manufacturing reduces downtime enhances productivity and improves product quality for a competitive edge.
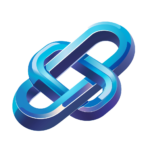
Predictive Maintenance in Food Manufacturing: AI’s Role in Reducing Downtime
Understanding Predictive Maintenance
Predictive maintenance is a proactive approach that leverages data analytics and machine learning to predict equipment failures before they occur. In the food manufacturing sector, where operational efficiency is paramount, this strategy can significantly reduce downtime, enhance productivity, and ensure product quality. By integrating artificial intelligence (AI) into predictive maintenance, food manufacturers can harness real-time data to make informed decisions and optimize their maintenance schedules.
The Importance of AI in Food Manufacturing
AI technologies are transforming food manufacturing by enabling smarter operations. The integration of AI into predictive maintenance processes allows companies to monitor equipment health continuously, analyze historical data, and predict potential failures. This shift not only minimizes unplanned downtime but also extends the lifespan of machinery, ultimately leading to cost savings and increased operational efficiency.
Key Benefits of AI-Driven Predictive Maintenance
- Reduced Downtime: By predicting failures before they happen, manufacturers can schedule maintenance during non-peak hours, reducing production interruptions.
- Cost Savings: Preventative measures can be more cost-effective than reactive repairs, significantly lowering maintenance costs.
- Improved Safety: Regular monitoring and maintenance reduce the risk of equipment-related accidents, ensuring a safer work environment.
- Enhanced Product Quality: Well-maintained equipment is less likely to produce defective products, maintaining high standards of quality.
Implementing AI in Predictive Maintenance
To effectively implement AI-driven predictive maintenance in food manufacturing, organizations must consider several steps:
1. Data Collection
Begin with the collection of data from various sources, including machinery sensors, maintenance logs, and production data. This data serves as the foundation for AI algorithms to analyze and identify patterns.
2. Data Analysis
Utilize machine learning algorithms to analyze the collected data. These algorithms can identify trends, predict potential failures, and provide insights into equipment performance.
3. Tool Selection
Select AI-driven tools that align with your operational needs. Some notable examples include:
- IBM Maximo: This enterprise asset management solution utilizes AI to predict equipment failures and optimize maintenance schedules.
- Predictive Maintenance by Siemens: This tool combines IoT data with AI analytics to enhance predictive maintenance capabilities in manufacturing environments.
- Uptake: A data analytics platform that provides actionable insights and predictive maintenance recommendations for industrial equipment.
- GE Digital’s Predix: This platform uses machine learning to analyze data from industrial equipment, helping manufacturers predict failures and optimize operations.
4. Continuous Monitoring and Improvement
Once AI tools are implemented, continuous monitoring is essential. Regularly assess the performance of the predictive maintenance system and make adjustments as necessary to improve accuracy and effectiveness.
Case Studies: Success Stories in Predictive Maintenance
Several food manufacturing companies have successfully implemented AI-driven predictive maintenance strategies:
Case Study 1: Nestlé
Nestlé adopted AI-powered predictive maintenance to monitor its production lines. By analyzing data from machinery sensors, the company was able to predict equipment failures, resulting in a 30% reduction in unplanned downtime.
Case Study 2: Tyson Foods
Tyson Foods implemented a predictive maintenance program using AI analytics to optimize its processing equipment. This initiative not only improved operational efficiency but also led to significant cost savings in maintenance expenses.
Conclusion
In an industry where efficiency and quality are critical, predictive maintenance powered by AI presents a transformative opportunity for food manufacturers. By adopting AI-driven tools and strategies, companies can significantly reduce downtime, enhance product quality, and achieve substantial cost savings. As the food manufacturing landscape continues to evolve, embracing these innovations will be essential for maintaining a competitive edge.
Keyword: AI predictive maintenance food manufacturing