AI in Predictive Maintenance for Reducing Utility Downtime
Topic: AI Data Tools
Industry: Energy and Utilities
Discover how AI enhances predictive maintenance in utilities to reduce downtime optimize operations and improve service delivery for better customer satisfaction
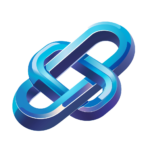
Predictive Maintenance: AI’s Role in Reducing Utility Downtime
Understanding Predictive Maintenance
Predictive maintenance refers to the use of advanced analytics and artificial intelligence (AI) to predict when equipment failures might occur, allowing organizations to perform maintenance before a failure happens. This proactive approach is particularly vital in the utilities sector, where downtime can lead to significant operational disruptions and financial losses.
The Importance of AI in Utilities
In the energy and utilities industry, maintaining operational continuity is paramount. Traditional maintenance practices often rely on scheduled checks or reactive strategies, which can be inefficient and costly. By integrating AI into predictive maintenance strategies, utilities can leverage data-driven insights to optimize their operations.
How AI Enhances Predictive Maintenance
AI enhances predictive maintenance through various methodologies, including machine learning algorithms, data analytics, and real-time monitoring. These technologies allow for the analysis of vast amounts of data generated by utility infrastructure, such as sensors and smart meters. The insights derived from this data can help identify patterns and anomalies that signal potential equipment failures.
Implementation of AI in Predictive Maintenance
Implementing AI for predictive maintenance involves several key steps:
- Data Collection: Utilities must first gather data from various sources, including operational logs, equipment sensors, and historical maintenance records.
- Data Analysis: Advanced analytics tools are employed to process and analyze the collected data, identifying trends and potential failure points.
- Model Development: Machine learning models are developed to predict equipment failures based on the analyzed data.
- Integration: These models are then integrated into the utility’s operational framework, enabling real-time monitoring and alerts.
Examples of AI-Driven Tools for Utilities
Several AI-driven products and tools are available that can significantly enhance predictive maintenance efforts in the utilities sector:
IBM Maximo
IBM Maximo is an asset management platform that utilizes AI to support predictive maintenance initiatives. By analyzing historical data and real-time sensor inputs, Maximo can forecast equipment failures and recommend maintenance schedules, thereby reducing unplanned downtime.
GE Digital’s Predix
GE Digital’s Predix platform is designed specifically for industrial applications, including utilities. It employs machine learning algorithms to analyze operational data and provide actionable insights for predictive maintenance, optimizing asset performance and extending equipment lifespan.
Siemens MindSphere
Siemens MindSphere offers a cloud-based IoT operating system that integrates AI capabilities for predictive maintenance. It allows utilities to connect their assets and analyze data, enabling them to predict maintenance needs and improve overall operational efficiency.
Benefits of AI-Driven Predictive Maintenance
The benefits of implementing AI-driven predictive maintenance in utilities are manifold:
- Reduced Downtime: By predicting failures before they occur, utilities can minimize downtime and maintain service continuity.
- Cost Savings: Proactive maintenance reduces the costs associated with emergency repairs and unplanned outages.
- Improved Safety: Predictive maintenance helps ensure that equipment operates safely, reducing the risk of accidents and injuries.
- Enhanced Efficiency: AI-driven insights enable utilities to optimize their maintenance schedules and resource allocation.
Conclusion
As the energy and utilities sector continues to evolve, the integration of AI into predictive maintenance strategies will play a crucial role in enhancing operational efficiency and reducing downtime. By leveraging advanced analytics and machine learning, utilities can not only predict equipment failures but also transform their maintenance practices, leading to improved service delivery and customer satisfaction.
Keyword: AI predictive maintenance utilities