AI in Predictive Maintenance for Textile Manufacturing Benefits
Topic: AI Fashion Tools
Industry: Textile Manufacturing
Discover how AI-driven predictive maintenance transforms textile manufacturing by reducing downtime costs and enhancing operational efficiency for a competitive edge
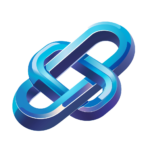
Predictive Maintenance in Textile Manufacturing: AI’s Game-Changing Role
Understanding Predictive Maintenance
Predictive maintenance refers to the proactive approach of using data analytics to predict when equipment failures might occur. In the textile manufacturing industry, where machinery plays a critical role in production efficiency, implementing predictive maintenance can significantly reduce downtime and operational costs. By leveraging artificial intelligence (AI), manufacturers can analyze vast amounts of data from their machines to forecast potential issues before they escalate into costly breakdowns.
The Role of AI in Predictive Maintenance
Artificial intelligence enhances predictive maintenance by enabling real-time monitoring and data analysis. AI algorithms can process historical data, identify patterns, and predict equipment failures with remarkable accuracy. This not only helps in scheduling timely maintenance but also aids in optimizing the overall production workflow.
Key AI Technologies in Predictive Maintenance
Several AI technologies are pivotal in facilitating predictive maintenance in textile manufacturing:
- Machine Learning: Machine learning algorithms can analyze historical performance data to identify anomalies and predict when maintenance should be performed. For example, a textile manufacturer might use machine learning to analyze vibration data from weaving machines to detect irregular patterns indicative of wear.
- IoT Sensors: Internet of Things (IoT) sensors can be integrated into textile machinery to collect real-time data on performance metrics such as temperature, pressure, and operational speed. This data can be fed into AI systems for analysis.
- Predictive Analytics: Predictive analytics tools can forecast potential failures by utilizing data from various sources, including machine logs and maintenance records. This enables manufacturers to transition from reactive to proactive maintenance strategies.
Implementing AI-Driven Predictive Maintenance Tools
To effectively implement predictive maintenance, textile manufacturers can utilize various AI-driven tools and products. Here are a few noteworthy examples:
1. IBM Maximo
IBM Maximo is an asset management platform that employs AI to enhance predictive maintenance capabilities. It allows textile manufacturers to monitor equipment health in real-time, analyze historical data, and predict failures. By integrating IoT data, Maximo can provide actionable insights to optimize maintenance schedules.
2. Siemens MindSphere
Siemens MindSphere is a cloud-based IoT operating system that connects industrial machines and systems. It provides advanced analytics tools that can help textile manufacturers monitor machine performance and predict maintenance needs. By utilizing MindSphere, companies can reduce unplanned downtime and improve overall equipment effectiveness.
3. GE Digital’s Predix
Predix is a platform designed for industrial IoT applications, facilitating predictive maintenance through data analytics. Textile manufacturers can use Predix to analyze machine data, predict failures, and implement maintenance strategies that reduce costs and enhance productivity.
4. TensorFlow
TensorFlow, an open-source machine learning framework, can be utilized to develop custom predictive maintenance models tailored to specific machinery in textile manufacturing. By training models on historical data, manufacturers can create highly accurate predictions regarding equipment health and maintenance needs.
Benefits of AI-Driven Predictive Maintenance
The integration of AI in predictive maintenance offers numerous benefits for textile manufacturers:
- Reduced Downtime: By predicting failures before they occur, manufacturers can schedule maintenance during non-peak hours, minimizing production interruptions.
- Cost Savings: Proactive maintenance reduces the costs associated with emergency repairs and unplanned downtime, leading to significant savings over time.
- Increased Equipment Lifespan: Regularly scheduled maintenance based on predictive analytics can extend the life of machinery, ensuring consistent production quality.
- Improved Operational Efficiency: Streamlined maintenance processes contribute to a more efficient production line, enhancing overall operational performance.
Conclusion
In the rapidly evolving textile manufacturing landscape, the adoption of AI-driven predictive maintenance is not just an option; it is becoming a necessity. By leveraging advanced technologies such as machine learning, IoT, and predictive analytics, manufacturers can significantly enhance their operational efficiency, reduce costs, and maintain a competitive edge. As the industry continues to embrace digital transformation, those who invest in AI-driven predictive maintenance tools will undoubtedly reap the benefits of improved productivity and sustainability.
Keyword: AI predictive maintenance textile manufacturing