AI in Predictive Maintenance Reduces Utility Downtime Effectively
Topic: AI Research Tools
Industry: Energy and Utilities
Discover how AI-driven predictive maintenance reduces utility downtime by forecasting equipment failures and optimizing maintenance schedules for enhanced efficiency
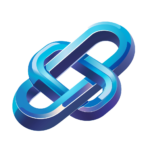
Predictive Maintenance: AI’s Role in Reducing Utility Downtime
Understanding Predictive Maintenance
Predictive maintenance is a proactive approach that leverages data analytics and machine learning to forecast equipment failures before they occur. In the energy and utilities sector, where downtime can lead to significant financial losses and service disruptions, the implementation of predictive maintenance strategies is crucial. By analyzing historical data and real-time sensor information, organizations can identify patterns and anomalies that indicate potential issues, allowing for timely interventions.
The Role of Artificial Intelligence in Predictive Maintenance
Artificial intelligence (AI) plays a pivotal role in enhancing predictive maintenance efforts. By utilizing advanced algorithms and machine learning techniques, AI can process vast amounts of data more efficiently than traditional methods. This capability enables utilities to not only predict failures but also optimize maintenance schedules, ultimately reducing operational costs and improving service reliability.
Key AI Technologies in Predictive Maintenance
Several AI-driven technologies are making significant strides in predictive maintenance within the energy and utilities sector:
1. Machine Learning Algorithms
Machine learning algorithms can analyze historical performance data to identify trends and predict future equipment failures. For instance, utilities can use supervised learning models to classify equipment health states based on sensor readings, enabling maintenance teams to prioritize interventions.
2. Natural Language Processing (NLP)
NLP can be utilized to analyze maintenance logs and reports, extracting valuable insights about equipment performance and common failure modes. This information can inform predictive models and help technicians focus on the most critical issues.
3. Internet of Things (IoT)
The integration of IoT devices allows for real-time monitoring of equipment conditions. Sensors can provide continuous data on temperature, vibration, and other critical parameters, which AI algorithms can analyze to detect anomalies indicative of impending failures.
Examples of AI-Driven Tools and Products
Several AI-driven tools and products are currently available that facilitate predictive maintenance in the energy and utilities sector:
1. IBM Maximo
IBM Maximo is an asset management platform that incorporates AI and machine learning to optimize maintenance operations. The software provides predictive insights based on historical data, enabling utilities to schedule maintenance activities more effectively and minimize downtime.
2. GE Digital’s Predix
GE Digital’s Predix platform is designed for industrial IoT applications and offers robust analytics capabilities. By harnessing data from connected assets, Predix helps utilities predict failures and optimize asset performance, significantly reducing unplanned outages.
3. Siemens MindSphere
Siemens MindSphere is a cloud-based IoT operating system that utilizes advanced analytics and machine learning to enhance predictive maintenance strategies. It allows utilities to connect their equipment, collect data, and gain actionable insights that lead to improved operational efficiency.
Implementing AI for Predictive Maintenance
To successfully implement AI-driven predictive maintenance solutions, utilities should consider the following steps:
1. Data Collection and Integration
Establish a robust data collection framework that integrates data from various sources, including sensors, maintenance logs, and historical performance records. This comprehensive data set is essential for training AI models.
2. Choosing the Right AI Tools
Select AI tools that align with organizational goals and technical capabilities. Consider factors such as scalability, ease of integration, and the specific features that cater to predictive maintenance needs.
3. Continuous Monitoring and Improvement
After implementation, continuously monitor the performance of AI-driven predictive maintenance systems. Use feedback loops to refine algorithms and improve predictive accuracy, ensuring that the system evolves with changing operational conditions.
Conclusion
Predictive maintenance powered by artificial intelligence presents a transformative opportunity for the energy and utilities sector. By leveraging advanced analytics and machine learning, organizations can significantly reduce downtime, enhance operational efficiency, and provide reliable services to customers. As AI technologies continue to evolve, the potential for predictive maintenance will only expand, making it an essential strategy for utilities aiming to thrive in a competitive landscape.
Keyword: Predictive maintenance AI solutions