AI in Real Estate Lending Fraud Detection for Banks and Borrowers
Topic: AI Real Estate Tools
Industry: Banks and Financial Institutions
Discover how AI enhances fraud detection in real estate lending protecting banks and borrowers from risks while improving compliance and customer experience
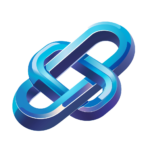
AI and Fraud Detection in Real Estate Lending: Protecting Banks and Borrowers
Introduction to AI in Real Estate Lending
The real estate lending sector has witnessed a transformative shift with the integration of artificial intelligence (AI) technologies. As banks and financial institutions navigate the complexities of lending, AI emerges as a powerful ally in combating fraud, ensuring compliance, and enhancing customer experiences. By leveraging AI-driven tools, institutions can protect themselves and their borrowers from the risks associated with fraudulent activities.
Understanding Fraud in Real Estate Lending
Fraud in real estate lending can take various forms, including identity theft, income falsification, and property valuation manipulation. These fraudulent activities not only pose significant financial risks to banks but also jeopardize the trust and safety of borrowers. As the volume of real estate transactions continues to grow, so does the sophistication of fraud schemes, making it imperative for financial institutions to adopt advanced technologies for effective fraud detection.
The Role of AI in Fraud Detection
Artificial intelligence can analyze vast amounts of data at unprecedented speeds, identifying patterns and anomalies that may indicate fraudulent behavior. By implementing AI algorithms, banks can enhance their fraud detection capabilities, streamline their lending processes, and ultimately safeguard their operations and clients.
Key AI Technologies in Fraud Detection
Several AI technologies are particularly effective in the realm of fraud detection within real estate lending:
Machine Learning Algorithms
Machine learning algorithms can be trained to recognize fraudulent patterns by analyzing historical data. For instance, a bank might utilize a machine learning model to assess loan applications, flagging those with discrepancies in financial documentation or unusual transaction histories.
Natural Language Processing (NLP)
NLP can be employed to analyze unstructured data, such as emails and social media interactions, to detect potential fraud risks. By scanning communications for red flags, banks can gain insights into borrower intentions and behaviors that may not be apparent through traditional analysis.
Predictive Analytics
Predictive analytics tools can forecast potential risks by evaluating various factors, including borrower credit scores, market trends, and economic indicators. For example, a predictive model could alert a bank to a higher likelihood of default based on changing economic conditions, allowing for proactive measures to mitigate risk.
Examples of AI-Driven Tools for Banks
Several AI-driven products are now available to assist banks and financial institutions in their fraud detection efforts:
Fraud Detection Platforms
Platforms like FICO Falcon and SAS Fraud Management utilize AI to monitor transactions in real-time, analyzing behavior patterns and flagging suspicious activities. These tools provide banks with actionable insights, enabling them to respond swiftly to potential fraud attempts.
Document Verification Solutions
AI-powered document verification tools, such as Ocrolus and Verifi, can automate the process of validating borrower documents. By employing optical character recognition (OCR) and machine learning, these solutions can quickly identify inconsistencies and verify the authenticity of financial statements, tax returns, and other critical documents.
Risk Assessment Models
AI-driven risk assessment models, such as Zest AI, provide banks with enhanced credit scoring capabilities. By analyzing a broader range of variables beyond traditional credit scores, these models can offer a more comprehensive view of borrower risk, helping lenders make informed decisions while minimizing fraud exposure.
Conclusion
The integration of AI technologies in real estate lending represents a significant advancement in the fight against fraud. By adopting AI-driven tools, banks and financial institutions can enhance their fraud detection capabilities, protect their assets, and foster trust with borrowers. As the landscape of real estate lending continues to evolve, the strategic implementation of AI will be essential in navigating challenges and seizing opportunities for growth.
Keyword: AI fraud detection real estate