AI in Renewable Energy Forecasting Enhancing Efficiency and Reliability
Topic: AI News Tools
Industry: Energy and Utilities
Discover how AI is transforming renewable energy forecasting by enhancing accuracy and efficiency for a sustainable energy future. Explore key tools and strategies.
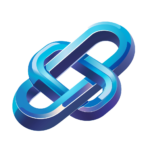
The Rise of AI in Renewable Energy Forecasting: Boosting Efficiency and Reliability
Understanding the Role of AI in Renewable Energy
The integration of artificial intelligence (AI) into renewable energy forecasting marks a significant evolution in how energy providers operate. With the increasing complexity of energy systems and the need for more reliable forecasting methods, AI has emerged as a critical tool in enhancing efficiency and reliability in the energy sector.
The Need for Accurate Forecasting
Accurate forecasting of renewable energy production is essential for optimizing grid operations and ensuring a stable energy supply. Traditional forecasting methods often struggle to account for the variability inherent in renewable sources such as solar and wind. AI technologies, however, offer sophisticated algorithms capable of analyzing vast datasets to improve prediction accuracy.
How AI Enhances Forecasting Accuracy
AI employs machine learning models that can learn from historical data, identify patterns, and make real-time predictions. By leveraging data from weather forecasts, historical energy production, and consumption patterns, AI systems can provide highly accurate forecasts that help energy providers manage supply and demand more effectively.
Key AI Tools and Products in Renewable Energy Forecasting
1. IBM Watson for Energy
IBM Watson for Energy utilizes advanced analytics and machine learning to enhance energy forecasting. By integrating weather data and historical performance metrics, it enables utilities to predict energy production from renewable sources with greater precision. This tool aids in optimizing energy dispatch and reducing operational costs.
2. Google DeepMind’s Wind Energy Prediction
Google DeepMind has developed AI algorithms specifically aimed at improving wind energy forecasting. By analyzing historical wind data and real-time weather information, this system can predict energy output up to 36 hours in advance, allowing operators to make informed decisions about energy distribution and storage.
3. Siemens’ Predictive Maintenance Solutions
Siemens offers predictive maintenance solutions that utilize AI to monitor the health of renewable energy assets. By analyzing performance data, these tools can forecast potential failures and maintenance needs, ensuring that energy production remains consistent and reliable.
4. Enel’s Advanced Analytics Platform
Enel has implemented an advanced analytics platform that leverages AI to enhance renewable energy forecasting. This platform integrates weather forecasts, satellite imagery, and grid data to provide real-time insights into energy generation capabilities, enabling better decision-making for energy distribution.
Implementing AI in Renewable Energy Forecasting
To successfully implement AI in renewable energy forecasting, organizations must consider several key factors:
1. Data Collection and Management
Robust data collection systems are essential for feeding AI algorithms. Companies need to invest in technologies that gather real-time data from multiple sources, including weather stations, sensors on renewable energy assets, and historical performance records.
2. Choosing the Right AI Tools
Selecting appropriate AI tools tailored to specific forecasting needs is crucial. Organizations should evaluate various products based on their capabilities, ease of integration, and scalability to ensure they meet operational demands.
3. Continuous Learning and Adaptation
AI models require continuous training and adaptation to remain effective. Energy providers should establish processes for regularly updating their algorithms with new data to enhance forecasting accuracy and reliability over time.
Conclusion
The rise of AI in renewable energy forecasting represents a transformative shift in the energy sector. By improving accuracy and efficiency, AI tools not only enhance operational reliability but also contribute to a more sustainable energy future. As the demand for renewable energy continues to grow, the integration of AI technologies will be pivotal in meeting global energy needs while minimizing environmental impacts.
Keyword: AI in renewable energy forecasting