AI Language Models in Medical Research Bias and Discovery Insights
Topic: AI Language Tools
Industry: Healthcare
Explore how AI language models are transforming medical research by accelerating discovery while addressing concerns about bias in healthcare outcomes.
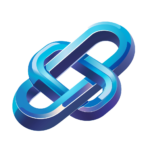
AI Language Models in Medical Research: Accelerating Discovery or Introducing Bias?
The Role of AI in Healthcare
Artificial Intelligence (AI) is transforming various sectors, and healthcare is no exception. Within this domain, AI language models are emerging as powerful tools, facilitating research, enhancing patient care, and improving operational efficiencies. However, the integration of these models raises critical questions regarding their impact on medical research, particularly concerning the potential for bias.
Understanding AI Language Models
AI language models, such as OpenAI’s GPT-3 and Google’s BERT, utilize machine learning algorithms to process and generate human-like text. These models can analyze vast amounts of medical literature, extract relevant information, and summarize findings, significantly accelerating the research process. For instance, researchers can leverage these tools to identify trends, generate hypotheses, and even draft research papers.
Examples of AI-Driven Tools in Medical Research
- IBM Watson for Health: This AI platform analyzes unstructured data from various sources, including clinical notes and research publications, to provide insights that can guide treatment decisions and accelerate drug discovery.
- Elsevier’s PharmaPendium: This tool uses AI to streamline the review of drug approval documents, making it easier for researchers to access critical information regarding drug efficacy and safety.
- DeepMind’s AlphaFold: While primarily focused on protein folding, AlphaFold employs AI to predict protein structures, which is vital for understanding diseases and developing new treatments.
Accelerating Discovery
One of the most significant advantages of AI language models is their ability to process and analyze large datasets quickly. Traditional research methods can be time-consuming and labor-intensive, often taking years to yield results. AI tools can expedite this process by automating data analysis and providing real-time insights.
Case Study: Drug Discovery
In drug discovery, AI language models can analyze clinical trial data and scientific literature to identify potential drug candidates more efficiently. For example, BenevolentAI utilizes AI to sift through millions of scientific papers and databases, enabling researchers to discover new applications for existing drugs or identify novel compounds for further investigation.
The Risk of Introducing Bias
Despite the many benefits, the deployment of AI language models in medical research is not without its challenges. One of the primary concerns is the potential for bias. AI systems learn from historical data, and if that data contains biases—whether related to race, gender, or socioeconomic status—these biases can be perpetuated in the AI’s outputs.
Examples of Bias in AI
For instance, if an AI model is trained predominantly on research involving a specific demographic, it may not perform as effectively when applied to underrepresented populations. This can lead to disparities in healthcare outcomes and hinder the development of equitable treatment protocols.
Mitigating Bias in AI Language Models
To harness the full potential of AI while minimizing bias, healthcare organizations must implement strategies that promote fairness and inclusivity in AI development. This includes:
- Diverse Training Data: Ensuring that AI models are trained on diverse datasets that represent various demographics can help reduce bias.
- Regular Audits: Conducting regular audits of AI systems to identify and address any biases in their outputs.
- Collaboration with Ethicists: Involving ethicists and social scientists in the development of AI tools can provide valuable insights into the ethical implications of AI in healthcare.
Conclusion
AI language models hold tremendous promise for accelerating medical research and improving patient outcomes. However, it is imperative for stakeholders in the healthcare sector to remain vigilant about the potential for bias. By implementing robust strategies to mitigate bias and promote diversity in AI training data, the healthcare industry can leverage these powerful tools responsibly and effectively.
Keyword: AI language models in healthcare