AI Pest Detection Networks for Effective Crop Protection
Topic: AI Networking Tools
Industry: Agriculture
Discover how AI-enabled pest detection networks enhance crop protection with early warning systems for timely pest management and sustainable farming practices.
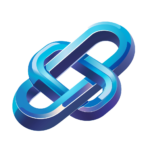
AI-Enabled Pest Detection Networks: Early Warning Systems for Crop Protection
Introduction to AI in Agriculture
The integration of artificial intelligence (AI) into agriculture has revolutionized traditional farming practices, providing farmers with innovative tools to enhance productivity and sustainability. One of the most promising applications of AI in this sector is the development of AI-enabled pest detection networks, which serve as early warning systems for crop protection. These systems leverage advanced data analytics, machine learning, and real-time monitoring to identify pest threats before they can cause significant damage.
The Importance of Early Pest Detection
Pest infestations can lead to substantial losses in crop yield and quality, making timely detection critical for farmers. Traditional pest management strategies often rely on periodic inspections and historical data, which may not accurately reflect current pest activity. AI-enabled pest detection networks address this challenge by providing continuous monitoring and rapid response capabilities, enabling farmers to act swiftly to mitigate threats.
How AI Can Be Implemented in Pest Detection
AI can be implemented in pest detection through various methodologies, including image recognition, data analytics, and predictive modeling. By utilizing machine learning algorithms, these systems can analyze vast amounts of data from multiple sources, such as sensors, drones, and satellite imagery, to detect pest presence and predict potential outbreaks.
Key Components of AI-Enabled Pest Detection Networks
- Sensors and IoT Devices: These devices collect real-time environmental data, including temperature, humidity, and soil conditions, which are crucial for understanding pest behavior.
- Image Recognition Software: Advanced algorithms can analyze images captured by drones or cameras to identify pests at various life stages, allowing for precise targeting of pest management efforts.
- Data Analytics Platforms: These platforms aggregate data from different sources, providing farmers with actionable insights and trends related to pest activity.
- Predictive Analytics: By employing historical data and machine learning models, farmers can forecast pest outbreaks and implement preventative measures before infestations occur.
Examples of AI-Driven Tools for Pest Detection
1. CropX
CropX is an innovative platform that utilizes soil sensors and AI-driven analytics to provide farmers with insights into soil health and moisture levels. By understanding these parameters, farmers can better predict pest activity and optimize their pest management strategies accordingly.
2. PestNet
PestNet is a global network that leverages AI to identify and monitor pest outbreaks. Farmers can upload images of pests, which are then analyzed by the system to provide identification and management recommendations. This collaborative approach enhances pest detection accuracy and fosters knowledge sharing among farmers.
3. Taranis
Taranis combines aerial imagery with AI-powered analytics to monitor crop health and detect pests. The platform offers farmers the ability to visualize pest threats in real-time, enabling them to take targeted action based on precise data.
4. Plantix
Plantix is a mobile application that uses image recognition to diagnose plant diseases and pest infestations. Farmers can take pictures of affected crops, and the app provides instant feedback and recommendations for treatment, making it an invaluable tool for early intervention.
Conclusion
As the agricultural industry faces increasing challenges from pests and diseases, AI-enabled pest detection networks offer a proactive approach to crop protection. By leveraging advanced technologies, farmers can enhance their pest management strategies, reduce losses, and promote sustainable farming practices. The future of agriculture lies in the effective integration of AI tools, which will empower farmers to make data-driven decisions and safeguard their crops against emerging threats.
Keyword: AI pest detection systems