AI Powered Anti Money Laundering Tools for Financial Security
Topic: AI Research Tools
Industry: Finance and Banking
Discover how AI-powered anti-money laundering tools enhance detection streamline compliance and protect financial institutions from evolving financial crimes
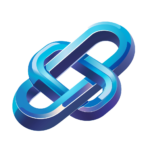
AI-Powered Anti-Money Laundering Tools: Staying Ahead of Financial Crime
Understanding the Role of AI in Financial Crime Prevention
In an era where financial transactions are increasingly digitized, the risk of money laundering and other financial crimes has surged. Traditional methods of detecting and preventing these illicit activities often fall short, leading to significant financial losses and reputational damage for institutions. However, the integration of artificial intelligence (AI) into anti-money laundering (AML) frameworks presents a transformative solution that not only enhances detection capabilities but also streamlines compliance processes.
How AI Can Be Implemented in AML Strategies
AI can be effectively implemented in AML strategies through various methodologies, including machine learning, natural language processing, and data analytics. These technologies enable financial institutions to analyze vast amounts of data in real-time, identify patterns indicative of money laundering, and reduce false positives that often plague traditional systems.
Machine Learning Algorithms
Machine learning algorithms can be trained on historical transaction data to identify anomalies that may suggest money laundering activities. By continuously learning from new data, these algorithms improve their predictive accuracy over time. For example, if a bank notices unusual transaction patterns from a specific account, its machine learning model can flag this activity for further investigation.
Natural Language Processing
Natural language processing (NLP) can enhance AML efforts by analyzing unstructured data, such as customer communications and social media interactions. By employing NLP, institutions can assess the sentiment and context of communications to identify potential risks associated with clients or transactions.
Examples of AI-Driven AML Tools
Several AI-powered tools are currently leading the charge in the fight against financial crime. Below are a few notable examples:
1. SAS Anti-Money Laundering
SAS offers a comprehensive AML solution that utilizes machine learning to detect suspicious activity across various channels. Its advanced analytics capabilities allow institutions to monitor transactions in real-time, providing insights that help compliance teams respond swiftly to potential threats.
2. Actico Compliance Suite
The Actico Compliance Suite integrates machine learning algorithms to automate the monitoring of transactions and customer behavior. Its flexible configuration allows institutions to tailor the system to their specific risk profiles, ensuring that they can adapt to evolving threats.
3. FICO TONBELLER
FICO TONBELLER employs AI-driven analytics to enhance transaction monitoring and customer due diligence processes. The system’s ability to adapt to changing patterns in financial crime ensures that institutions remain vigilant against emerging threats.
Benefits of AI-Driven AML Solutions
The adoption of AI-powered AML tools offers numerous advantages for financial institutions:
Enhanced Detection Rates
AI systems can analyze vast datasets far beyond human capabilities, significantly improving detection rates for suspicious transactions.
Reduced Operational Costs
By automating routine compliance tasks, AI tools can reduce the workload on compliance teams, allowing them to focus on more complex investigations.
Improved Regulatory Compliance
AI-driven solutions help institutions stay compliant with ever-evolving regulations by providing real-time insights and reporting capabilities, thus minimizing the risk of penalties.
Conclusion
As financial crime continues to evolve, so must the strategies employed to combat it. AI-powered anti-money laundering tools are not just a trend; they represent a critical advancement in the fight against financial crime. By integrating these technologies, financial institutions can enhance their detection capabilities, streamline compliance processes, and ultimately protect their assets and reputation. Embracing AI in AML is not merely an option but a necessity for staying ahead in today’s complex financial landscape.
Keyword: AI powered anti money laundering tools