AI Predictive Analytics for Energy Market Trend Forecasting
Topic: AI Finance Tools
Industry: Energy and Utilities
Discover how predictive analytics and AI can transform the energy sector by forecasting market trends optimizing operations and enhancing profitability
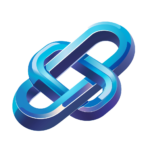
Predictive Analytics: Using AI to Anticipate Energy Market Trends
Understanding Predictive Analytics in the Energy Sector
Predictive analytics has emerged as a pivotal tool in the energy and utilities sector, leveraging artificial intelligence (AI) to forecast market trends and optimize operations. By analyzing historical data and identifying patterns, organizations can make informed decisions that enhance efficiency and profitability. This article explores how AI-driven predictive analytics can be implemented within the energy market and highlights specific tools that facilitate these advancements.
The Role of AI in Energy Market Forecasting
Artificial intelligence plays a crucial role in transforming vast amounts of data into actionable insights. By utilizing machine learning algorithms, AI can process complex datasets that encompass consumption patterns, weather forecasts, and economic indicators. This capability allows energy companies to anticipate fluctuations in demand and supply, ultimately leading to better resource allocation and strategic planning.
Key Applications of Predictive Analytics
1. Demand Forecasting: AI algorithms can analyze historical consumption data alongside external variables, such as temperature changes and economic activity, to predict energy demand accurately. This enables utilities to optimize generation and reduce operational costs.
2. Price Forecasting: Predictive analytics can also be employed to forecast energy prices based on market trends and historical pricing data. This information is invaluable for energy traders and financial analysts who seek to capitalize on market movements.
3. Risk Management: By predicting potential disruptions in supply chains or regulatory changes, AI tools can help organizations mitigate risks and develop contingency plans, ensuring operational resilience.
Examples of AI-Driven Tools in Energy Predictive Analytics
Several AI-driven products are currently available that cater to the unique needs of the energy and utilities sector. Here are a few notable examples:
1. IBM Watson
IBM Watson offers advanced analytics capabilities that can be tailored for the energy sector. Its machine learning models can analyze data from various sources to predict energy demand and optimize grid management. The platform’s natural language processing capabilities also allow users to interact with data in a more intuitive manner.
2. Uplight
Uplight provides a suite of AI-powered tools designed specifically for utilities. Their predictive analytics solutions help energy providers understand customer behavior, forecast demand, and enhance energy efficiency programs. Uplight’s platform also integrates seamlessly with existing utility systems, making it easier for organizations to adopt AI technologies.
3. Enel X
Enel X leverages AI to offer predictive analytics solutions that assist in demand response and energy management. Their tools analyze real-time data to forecast energy usage patterns, enabling businesses to optimize their energy consumption and reduce costs. Enel X’s platform is particularly beneficial for commercial and industrial clients seeking to enhance sustainability practices.
Implementing AI in Predictive Analytics
To successfully implement AI-driven predictive analytics in the energy sector, organizations should follow a structured approach:
- Data Collection: Gather relevant data from various sources, including smart meters, weather forecasts, and market reports.
- Data Integration: Ensure that data from different systems can be integrated and analyzed cohesively.
- Model Development: Develop machine learning models tailored to specific forecasting needs, using historical data to train the algorithms.
- Continuous Improvement: Regularly update models with new data to enhance accuracy and adapt to changing market conditions.
Conclusion
As the energy market continues to evolve, the integration of predictive analytics powered by artificial intelligence will be critical for organizations seeking to maintain a competitive edge. By effectively leveraging AI tools, energy companies can anticipate market trends, optimize operations, and ultimately drive greater profitability. Embracing these technologies not only enhances decision-making processes but also fosters a more sustainable energy future.
Keyword: AI predictive analytics energy market