AI Predictive Analytics Transforming CPG Supply Chain Management
Topic: AI Marketing Tools
Industry: Consumer Packaged Goods (CPG)
Discover how AI-driven predictive analytics is revolutionizing CPG supply chain management by enhancing efficiency and meeting consumer demands in real-time
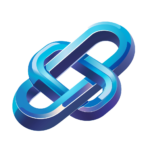
AI-Driven Predictive Analytics: The Future of CPG Supply Chain Management
Understanding the Role of Predictive Analytics in CPG
In the fast-paced world of Consumer Packaged Goods (CPG), the ability to anticipate market trends and consumer preferences is paramount. Predictive analytics, powered by artificial intelligence (AI), offers CPG companies the tools necessary to make data-driven decisions that enhance supply chain efficiency and responsiveness. By leveraging historical data, market trends, and consumer behavior, businesses can forecast demand, optimize inventory levels, and reduce waste.
Implementing AI in CPG Supply Chain Management
Integrating AI-driven predictive analytics into supply chain management involves several key steps:
1. Data Collection and Integration
The first step is to gather relevant data from various sources, including sales data, customer feedback, market research, and social media trends. This data must be integrated into a centralized system to provide a comprehensive view of the market landscape.
2. Choosing the Right AI Tools
Several AI-driven tools can facilitate predictive analytics in the CPG sector. Some notable examples include:
- SAS Demand Forecasting: This tool uses advanced algorithms to analyze historical sales data and predict future demand, enabling companies to optimize their inventory levels.
- IBM Watson Supply Chain: Leveraging machine learning, this platform provides insights into supply chain risks and opportunities, helping businesses make informed decisions.
- Microsoft Azure Machine Learning: This cloud-based service allows CPG companies to build, train, and deploy machine learning models for demand forecasting and inventory management.
3. Model Development and Testing
After selecting the appropriate tools, businesses must develop predictive models tailored to their specific needs. This involves training the models using historical data and continuously testing them against real-time data to ensure accuracy and reliability.
4. Implementation and Continuous Improvement
Once the models are developed, they can be integrated into existing supply chain processes. Continuous monitoring and refinement of these models are essential to adapt to changing market conditions and consumer behaviors.
Real-World Applications of AI-Driven Predictive Analytics
Several CPG companies have successfully implemented AI-driven predictive analytics to enhance their supply chain management:
Case Study: Procter & Gamble
Procter & Gamble (P&G) has utilized AI to optimize its supply chain by predicting demand fluctuations. By analyzing consumer behavior and market trends, P&G has improved its inventory management, resulting in reduced stockouts and improved customer satisfaction.
Case Study: Unilever
Unilever has harnessed AI-driven analytics to streamline its supply chain operations. By using machine learning algorithms to forecast demand, the company has minimized waste and improved its overall operational efficiency, leading to significant cost savings.
The Future of CPG Supply Chain Management
As AI technology continues to evolve, the potential for predictive analytics in CPG supply chain management will only grow. Companies that adopt these tools will gain a competitive edge, enabling them to respond swiftly to market changes and consumer demands.
Conclusion
AI-driven predictive analytics is transforming the landscape of supply chain management in the CPG sector. By implementing advanced AI tools and continuously refining predictive models, companies can enhance their operational efficiency and better meet consumer needs. The future of CPG supply chain management lies in embracing these technologies and leveraging data to drive strategic decision-making.
Keyword: AI predictive analytics CPG supply chain