AI Predictive Analytics Transforming Drug Demand Forecasting 2025
Topic: AI Productivity Tools
Industry: Pharmaceuticals
Discover how AI-powered predictive analytics will transform drug demand forecasting in the pharmaceutical industry by 2025 enhancing efficiency and patient access
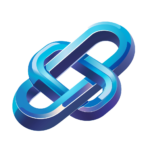
AI-Powered Predictive Analytics: Forecasting Drug Demand in 2025
The Growing Importance of Predictive Analytics in Pharmaceuticals
In the rapidly evolving pharmaceutical industry, the ability to accurately forecast drug demand is critical for optimizing supply chains, managing inventory, and ensuring that patients have access to necessary medications. As we approach 2025, the integration of artificial intelligence (AI) into predictive analytics is set to revolutionize how pharmaceutical companies anticipate market needs and respond to fluctuations in demand.Understanding Predictive Analytics
Predictive analytics involves using historical data, statistical algorithms, and machine learning techniques to identify the likelihood of future outcomes based on past events. In the context of pharmaceuticals, this means leveraging vast amounts of data—from clinical trials to market trends—to predict which drugs will be in demand and when.AI Implementation in Predictive Analytics
To effectively implement AI in predictive analytics, pharmaceutical companies must focus on several key areas: 1. Data Integration: Combining data from various sources—such as electronic health records, sales data, and social media—enables a comprehensive view of market dynamics. AI tools can automate this integration process, reducing the time and effort required to gather and analyze data. 2. Machine Learning Models: Developing robust machine learning models is essential for accurate forecasting. These models can learn from historical data patterns and adjust predictions based on new data inputs, providing real-time insights into drug demand. 3. Scenario Analysis: AI-powered tools can simulate different market scenarios, allowing companies to assess potential impacts of external factors such as regulatory changes, economic shifts, or emerging health trends.Specific AI-Driven Tools and Products
Several AI-driven tools and products are currently available that can enhance predictive analytics in the pharmaceutical sector: 1. IBM Watson Health: This platform utilizes AI to analyze vast datasets, providing insights into drug efficacy, market trends, and patient outcomes. By leveraging Watson’s capabilities, pharmaceutical companies can better predict demand for specific medications. 2. SAP Integrated Business Planning (IBP): This tool offers advanced analytics and machine learning capabilities to forecast demand more accurately. SAP IBP allows companies to create more agile supply chain strategies based on predictive insights. 3. Tableau with Einstein Analytics: Tableau, combined with Salesforce’s Einstein Analytics, provides a powerful solution for visualizing data and uncovering insights through AI-driven analytics. This tool can help pharmaceutical companies identify trends and make data-driven decisions regarding drug production and distribution. 4. Google Cloud AI: Google’s suite of AI tools can analyze complex datasets to identify patterns and predict future drug demand. Using Google Cloud’s machine learning capabilities, companies can enhance their forecasting accuracy and operational efficiency.Challenges and Considerations
While the benefits of AI-powered predictive analytics are substantial, pharmaceutical companies must also navigate several challenges: – Data Quality: The accuracy of predictions heavily relies on the quality of the data used. Ensuring that data is clean, relevant, and up-to-date is crucial for effective forecasting. – Regulatory Compliance: Adhering to regulatory standards is essential in the pharmaceutical industry. Companies must ensure that their AI tools comply with regulations regarding data privacy and security. – Change Management: Implementing AI solutions requires a cultural shift within organizations. Training staff and fostering an environment that embraces technological innovation is vital for successful adoption.Conclusion
As we look towards 2025, the integration of AI-powered predictive analytics in the pharmaceutical industry represents a significant advancement in how companies forecast drug demand. By leveraging advanced tools and technologies, pharmaceutical organizations can enhance their operational efficiency, reduce costs, and ultimately improve patient outcomes. Embracing these innovations will be essential for staying competitive in a dynamic market landscape.Keyword: AI predictive analytics pharmaceuticals