AI Predictive Analytics Transforming Food Quality Control
Topic: AI Cooking Tools
Industry: Food Safety and Quality Control
Discover how AI-powered predictive analytics is transforming food quality control by enhancing safety improving efficiency and maintaining consumer trust in the industry
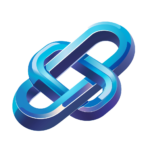
The Rise of AI-Powered Predictive Analytics in Food Quality Control
Understanding the Importance of Food Quality Control
In an era where consumer awareness regarding food safety and quality is at an all-time high, the food industry faces increasing pressure to ensure that products are not only safe but also of the highest quality. Food quality control is critical in preventing foodborne illnesses, maintaining brand reputation, and meeting regulatory standards. As a result, the integration of advanced technologies, particularly artificial intelligence (AI), has become a game-changer in this domain.
The Role of AI in Food Quality Control
Artificial intelligence has the potential to revolutionize food quality control processes by utilizing predictive analytics to forecast potential quality issues before they arise. By analyzing vast amounts of data from various sources, AI can identify patterns and anomalies that may indicate a decline in food quality or safety. This proactive approach not only enhances the efficiency of quality control measures but also significantly reduces the risk of costly recalls and consumer dissatisfaction.
Implementing AI-Powered Predictive Analytics
To effectively implement AI-powered predictive analytics in food quality control, organizations can adopt several strategies:
- Data Collection: Gather data from various stages of the food supply chain, including production, processing, and distribution. This data can include temperature logs, humidity levels, and even sensory data such as taste and smell.
- Machine Learning Algorithms: Utilize machine learning algorithms to analyze the collected data. These algorithms can identify trends and correlations that human analysts may overlook, providing deeper insights into potential quality issues.
- Real-time Monitoring: Implement real-time monitoring systems that leverage AI to continuously assess food quality. Such systems can alert operators to deviations from established quality parameters, allowing for immediate corrective actions.
Examples of AI-Driven Tools in Food Quality Control
Several innovative AI-driven products and tools are currently available that exemplify the application of predictive analytics in food quality control:
1. IBM Watson Food Safety
IBM Watson Food Safety utilizes AI to analyze data from various sources, including supply chain data, food safety regulations, and historical quality metrics. By employing natural language processing and machine learning, this platform can predict potential risks and suggest corrective actions, thereby enhancing food safety and quality compliance.
2. Pathogen Detection Systems
AI-powered pathogen detection systems, such as those developed by companies like BioMerieux, utilize machine learning to analyze microbial data and predict the likelihood of contamination in food products. These systems can significantly reduce the time required for testing, allowing for quicker decision-making and increased food safety.
3. Smart Sensors and IoT Devices
Smart sensors integrated with AI capabilities can monitor environmental conditions in real-time. For instance, devices like the FreshSurety system can monitor temperature and humidity levels throughout the supply chain. By analyzing this data, the system can predict potential spoilage and alert stakeholders to take preventive measures.
Challenges and Considerations
While the benefits of AI-powered predictive analytics in food quality control are substantial, several challenges must be addressed. Data privacy, integration with existing systems, and the need for skilled personnel to interpret AI-generated insights are key considerations. Additionally, organizations must ensure that AI systems are trained on high-quality data to avoid biases and inaccuracies in predictions.
Conclusion
The rise of AI-powered predictive analytics represents a significant advancement in food quality control. By embracing these technologies, food industry stakeholders can enhance safety, improve product quality, and maintain consumer trust. As AI continues to evolve, its role in food safety and quality control will undoubtedly expand, offering new opportunities for innovation and improvement in this critical sector.
Keyword: AI predictive analytics food quality