AI Predictive Maintenance for Energy Infrastructure Reduces Downtime
Topic: AI Communication Tools
Industry: Energy and Utilities
Discover how AI-driven predictive maintenance reduces downtime in energy infrastructure enhances efficiency and improves reliability for energy providers.
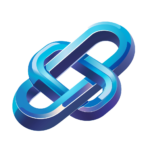
AI-Driven Predictive Maintenance: Reducing Downtime in Energy Infrastructure
The Role of Predictive Maintenance in Energy Infrastructure
In the ever-evolving landscape of energy and utilities, the need for efficient operations is paramount. Downtime not only leads to financial losses but can also impact service reliability and customer satisfaction. Predictive maintenance, powered by artificial intelligence (AI), emerges as a transformative solution to this challenge. By leveraging AI communication tools, organizations can proactively identify and address potential equipment failures before they occur, thereby minimizing interruptions and enhancing operational efficiency.
Understanding Predictive Maintenance
Predictive maintenance involves using data analytics to predict when equipment failure might occur. This approach contrasts with traditional maintenance strategies, which often rely on scheduled maintenance or reactive repairs. By implementing AI-driven predictive maintenance, energy infrastructure can transition from a reactive to a proactive maintenance model, significantly reducing downtime and associated costs.
How AI Enhances Predictive Maintenance
AI enhances predictive maintenance through several key mechanisms:
- Data Analysis: AI algorithms can analyze vast amounts of data from sensors and historical maintenance records to identify patterns and predict potential failures.
- Real-Time Monitoring: AI-driven tools enable real-time monitoring of equipment, allowing for immediate alerts when anomalies are detected.
- Decision Support: AI can provide actionable insights, helping maintenance teams prioritize tasks based on urgency and impact.
Implementing AI-Driven Predictive Maintenance
Organizations looking to implement AI-driven predictive maintenance can utilize a variety of tools and products designed specifically for the energy sector. Here are a few examples:
1. IBM Maximo
IBM Maximo is an asset management platform that integrates AI capabilities to enhance predictive maintenance. By analyzing data from IoT devices and historical maintenance records, Maximo can predict equipment failures and suggest optimal maintenance schedules.
2. GE Digital’s Predix
GE Digital’s Predix platform is tailored for industrial applications, providing advanced analytics and machine learning capabilities. Predix can analyze sensor data from energy infrastructure to predict failures and optimize maintenance schedules, significantly reducing unexpected downtime.
3. Siemens MindSphere
Siemens MindSphere is an open cloud-based IoT operating system that allows for the integration of AI-driven analytics. By connecting various industrial assets, MindSphere can provide insights into equipment performance and maintenance needs, facilitating predictive maintenance strategies across energy infrastructure.
4. Uptake
Uptake offers AI-driven analytics specifically designed for the energy and utilities sector. By utilizing machine learning algorithms, Uptake can identify patterns in equipment performance and predict maintenance needs, enabling organizations to act before failures occur.
Benefits of AI-Driven Predictive Maintenance
The implementation of AI-driven predictive maintenance in energy infrastructure offers several significant benefits:
- Reduced Downtime: By predicting equipment failures before they happen, organizations can significantly reduce unplanned downtime.
- Cost Savings: Proactive maintenance can lead to lower repair costs and extended equipment lifespan.
- Improved Safety: Predictive maintenance can enhance safety by reducing the likelihood of equipment failures that could pose risks to personnel.
- Enhanced Reliability: With fewer disruptions, energy providers can offer more reliable services to their customers.
Conclusion
AI-driven predictive maintenance represents a pivotal advancement for energy infrastructure, transforming how organizations manage their assets. By implementing sophisticated AI communication tools, companies can not only reduce downtime but also enhance overall operational efficiency. As the energy sector continues to embrace digital transformation, the integration of AI technologies will be essential for staying competitive and meeting the demands of a rapidly changing market.
Keyword: AI predictive maintenance energy infrastructure